Key Highlights
- Understanding the significance of data team roles is paramount in today’s data-driven business landscape.
- A cohesive data team comprises diverse experts, each playing a crucial role in driving business value from data.
- Data scientists, data engineers, and data analysts are core roles with distinct responsibilities.
- Building a successful data team requires identifying skill gaps, fostering collaboration, and investing in training.
- As technology rapidly evolves, data teams are at the forefront of adopting AI, machine learning, and agile structures.
Introduction
In today’s business world, data is very valuable, like gold. Companies that can collect, look at, and use data well have a big advantage over others. According to recent studies, businesses that leverage data analytics see a 5-6% increase in productivity and profitability compared to their peers who do not use data-driven decision-making. This shows why having a strong data team is important. A data team has skilled people in data science, business intelligence, and other areas. Their job is to turn raw data into actionable insights, making data assets a crucial component of their work. In fact, 73% of companies are now investing in data analytics to drive decision-making and improve business outcomes. These insights help to make smart business decisions.
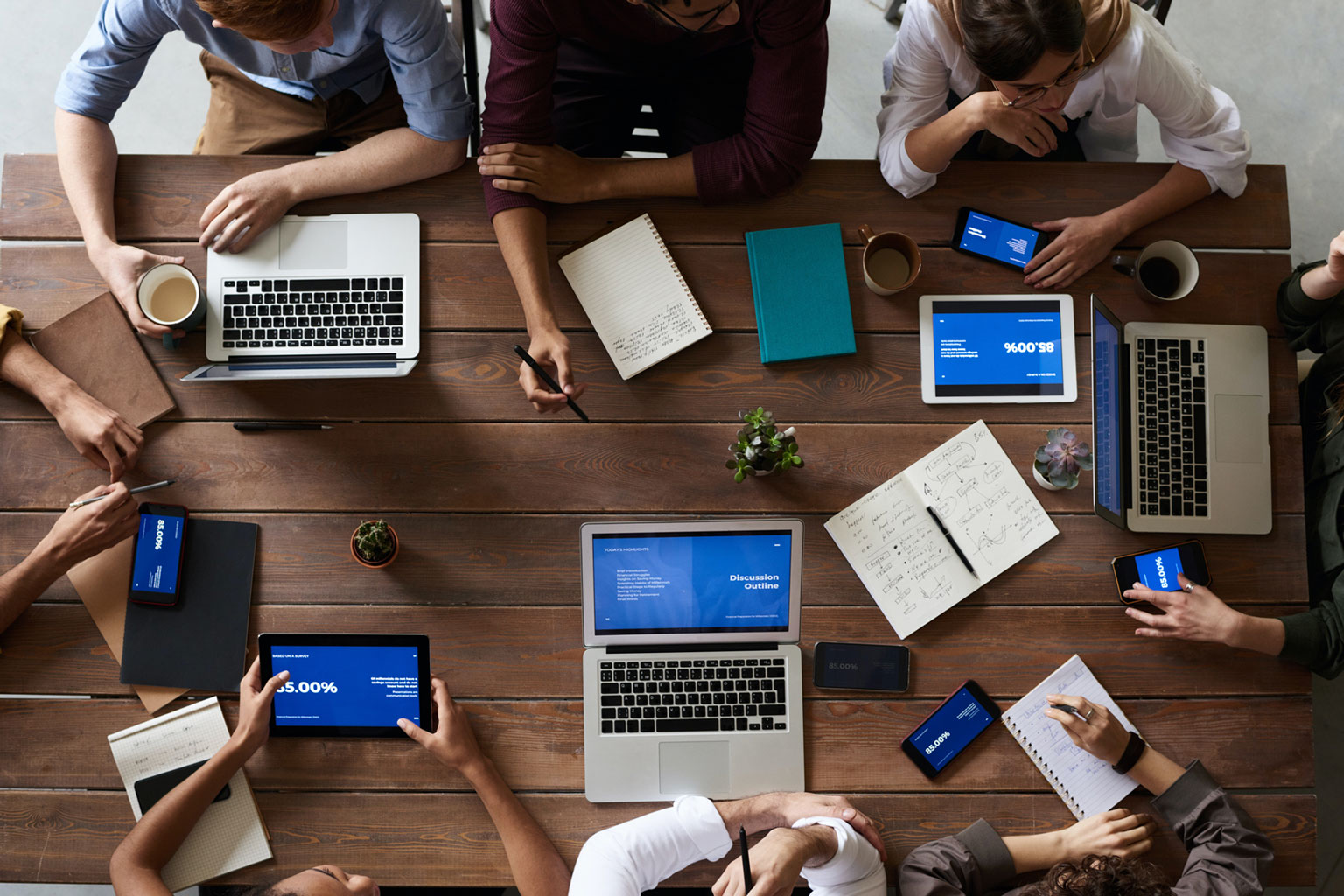
Understanding the Core of Data Teams in Modern Businesses
Data teams have changed from being something only big companies could have to being necessary for businesses of any size. They are key for making decisions based on data and use their skills to give organizations helpful insights. Research indicates that 92% of leading organizations are achieving measurable value from their data and analytics investments, underscoring the necessity of data teams for businesses. They help with many aspects, like improving marketing strategies, guessing what customers might do, and boosting how a business runs. With the increasing amount of data available, data management has become a crucial aspect of the role of data teams, specifically the data manager, in modern businesses. The global data management market is projected to reach $136.4 billion by 2026, highlighting the growing emphasis on data management.
By finding important patterns and trends in large amounts of data, these teams help understand what happened before, deal with what is happening now, and anticipate what might happen in the future.
Defining Data Team Dynamics and Their Evolution
The way a data science team works can change a lot. This often reflects new technology and the needs of the business. At first, data teams were put together in one main group. This main group helped the whole organization, but as companies grew, their data needs became more complex. This led to the rise of decentralized and hybrid models, making it crucial for larger organizations to adopt a hybrid model with a Center of Excellence to provide a data framework and individual business units to host their own data resources. Statistics show that 64% of companies are adopting hybrid models to better manage their data needs. Understanding the dynamics and evolution of data teams is essential for the success of larger organizations in utilizing data effectively.
In decentralized models, each business unit has its own data team. This approach helps them tackle specific data challenges better. The hybrid model combines both ideas. There is a central data team that creates broad strategies. At the same time, individual units have their own data professionals. This way, they can respond to their unique needs and utilize their data resources effectively. This change shows how important specialized data roles are and how they adapt to different business decisions.
The Impact of Data Teams on Business Strategy and Growth
The impact of data teams goes beyond just looking at numbers. They are key in forming business strategies and helping growth. With predictive analytics, data teams can predict future trends. This allows companies to make smart decisions ahead of time instead of waiting to react. It is estimated that businesses using predictive analytics have a 20% higher likelihood of outperforming their competitors.
For example, a retail company can use data analysis to improve its supply chain. By predicting changes in demand, they can make sure the right products are available when needed. This helps lower storage costs and boost sales. This way of using data leads to better inventory management, happier customers, and more profits. This shows the crucial role of data teams in driving business strategy and growth, as they are responsible for managing and analyzing data in a central location, known as a data warehouse, to provide valuable insights and inform decision-making processes.
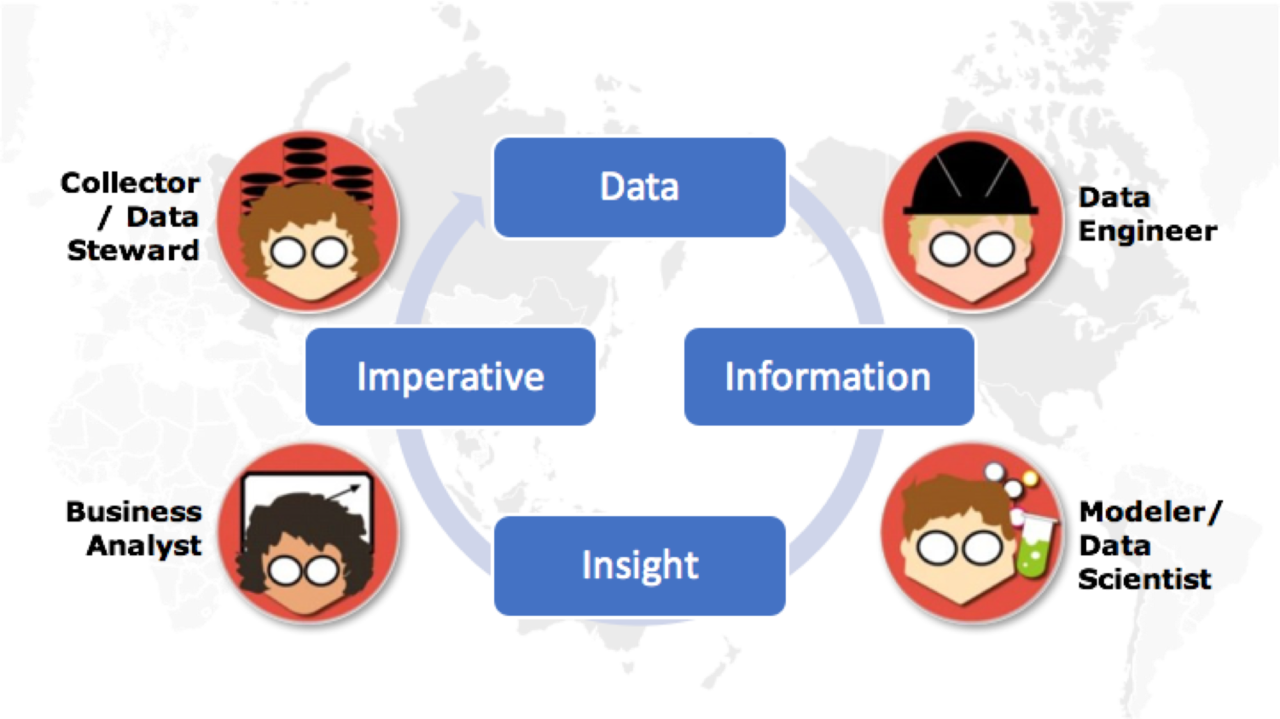
The Integral Roles Within a Data Team
A strong data team is made up of different roles. Each role has a special skill that is important for turning data into useful information. The specific roles can change based on how big the organization is and what industry it belongs to. However, some key roles stay the same in every data team, including the data analytics team. According to industry reports, 70% of companies have established dedicated data teams to harness the power of data effectively. Each role requires a high level of technical skill and is crucial for providing data insights to business users. Team members must also understand how to use data to solve business problems.
Let’s look at some of the important roles in a great data team.
The Role of Data Scientists in Gleaning Insights
Data scientists play a key role in a data team. They take raw data from the data pipeline and find useful insights. With the help of advanced analytics tools, they spot hidden patterns, trends, and connections that others might miss. They create statistical models and use machine learning techniques to predict future outcomes. It is estimated that 94% of businesses believe that data science is crucial to their growth and digital transformation. This helps businesses make informed decisions based on data, with the assistance of data visualization tools to present the findings in a clear and understandable manner.
Data scientists are also good at turning complex data into clear visuals and reports. This makes it easier for business leaders to understand and use the insights. Their skill in connecting raw data to actionable insights makes them vital to any data team.
How Data Engineers Facilitate Data Accessibility and Security
Data scientists look for useful information in data. On the other hand, data engineers build the system that makes data easy to access and use. They create and maintain the tools that allow for data collection, storage, processing, and analysis.
Data engineers also play an important role in keeping data safe. The demand for data engineers has increased by 50% in the past year, reflecting their critical role in data management. They set up protections to stop sensitive information from being stolen or misused. Their efforts create a strong and secure data environment, which is vital for any company that wants to make the most of its data.
The Crucial Function of Data Analysts in Business Decisions
Data analysts help connect technical information with business people. They change complicated data into useful insights that help make business decisions. They know how to ask questions in databases, do statistical analysis, and create visuals to show patterns and trends that everyone can understand. Data analysts are expected to see a job growth rate of 25% over the next decade, highlighting their growing importance in organizations.
Data analysts also team up with business managers. They listen to what managers need and turn that into detailed data questions and analyses. Their skill in sharing data in an interesting way makes them important for showing how data-based decisions affect everyone in the organization.
The Emerging Importance of Machine Learning Engineers
Machine learning is quickly changing many industries. Because of this, machine learning engineers are becoming very important for data teams. These experts create, build, and use machine learning models to make business processes better. Machine learning engineers are among the top emerging jobs, with a projected growth rate of 40% annually.
They know a lot about algorithms, data structures, and software engineering. Using this knowledge, they build predictive models and perform advanced deep learning workflows. These models can guess what customers will do, find unusual patterns, and make user experiences more personal. Their work helps big ideas grow and makes businesses run better. This shows how vital machine learning is for today’s data teams, making the role of machine learning engineers increasingly important.
Building a Cohesive Data Team: Best Practices
Building a strong data team is not just about hiring people with great skills. You need to have a plan. First, you should understand what your organization needs. Next, inspire teamwork and create a friendly culture. It’s also important to encourage learning all the time. A good team values different ideas and keeps communication open. This way, you can really use the power of data architecture and models. Reports indicate that nearly 80% of leaders recognize the importance of having the right people, processes, and culture to transform into a data-driven enterprise.
Here are some best practices to help you create and manage a successful data team.
Identifying and Filling Gaps in Your Data Team
The first step to building a strong data science team is to look closely at the skills gap. This means checking what skills the current team members have and comparing those to what the organization needs for data projects and future goals. After finding the gaps, you can create a focused plan for hiring.
Here are some important steps to take:
- Define Required Skill Sets: Clearly list the necessary technical and soft skills for each data role.
- Assess Existing Team Expertise: Check how skilled the current team members are in relation to the needed skills.
- Prioritize Skill Gaps: Figure out which missing skills are the most important for reaching short-term and long-term goals.
- Explore Recruitment Options: Look at promoting from within, hiring externally, or working with specialized agencies based on the urgency of the skill gap.
Fostering Collaboration Between Data Professionals and Business Units
A data team, no matter how skilled, cannot work alone. To find real business value, teamwork with different parts of the business is important. This helps make sure data projects match business goals, turns data insights into action, and lets the team’s work support the larger goals of the organization. Studies have shown that team cohesiveness can significantly predict positive outcomes in team collaboration, including satisfaction and goal attainment.
Organizations can promote teamwork by:
- Setting up clear ways to communicate: Have regular meetings, project updates, and feedback to help share information easily.
- Creating a shared understanding of goals: Make sure data professionals and business units agree on project goals, expectations, and measures of success.
- Supporting cross-functional projects: Give chances for data team members to collaborate with other parts of the business, building relationships and a better understanding of each other’s needs.
Training and Development for Data Team Excellence
The field of data science is always changing. New technologies and methods come up often. To keep up, organizations need to focus on ongoing training and professional development for their data teams.
Encouraging continuous learning can happen in different ways:
- Giving access to industry conferences and workshops: This helps team members stay informed about the latest trends, research, and best practices in data science.
- Supporting certifications and advanced degrees: Organizations can provide financial help and flexible scheduling for team members who want to get certifications or further education in data science.
- Hosting internal knowledge sharing sessions: This allows data professionals to share what they know and learn from their projects with the whole team, which helps create a culture of continuous learning.
Navigating the Challenges of Managing Data Teams
Managing a data team has its own challenges. You need to balance technical skills with business knowledge. It’s also important to ensure data is used ethically. Good communication among different team members is key. Data leaders must work hard to guide their teams to succeed, including taking data initiatives to prioritize growth opportunities and start relevant analytics projects. Facing these challenges directly is essential to build a strong data team that always produces meaningful results. According to a recent survey, 76% of organizations find it difficult to understand their data, and 82% cite controlling data costs as a major challenge, highlighting the complexities data teams face.
Balancing Technical Expertise with Business Acumen
Data workers often have strong technical skills. However, they may not fully understand the business context of their work. This gap can cause project misalignment, poor data interpretation, and lost chances.
Data leaders need to connect these areas by:
- Encouraging business knowledge: They should give team members chances to learn about the industry, products, and target market of the organization.
- Including business views in projects: It is important to make sure that project goals and data analysis match business aims and tackle real challenges.
- Supporting communication between data experts and business leaders: They should create chances for open talks and feedback to ensure everyone understands each other well.
Ensuring Data Privacy and Ethical Use in Projects
With more data being collected and studied, it’s important for data teams to focus on data privacy and ethics. An overwhelming majority of respondents (82%) cited confusing data governance policies as a top challenge, underscoring the importance of clear guidelines and governance. Organizations must create clear rules for data governance and guidelines that all team members should learn and follow.
Key points about using data ethically include:
- Data Privacy: Follow important data protection laws and use strong security to protect sensitive information.
- Bias Detection and Mitigation: Know that there can be biases in data sets and use methods to reduce these biases during analysis and model creation.
- Transparency and Accountability: Clearly explain how data is collected, how it’s analyzed, and the limits of the models to those who need to know.
Overcoming Communication Barriers Within Multidisciplinary Teams
Data teams have many people with different skills, backgrounds, and ways of talking. This mix can help solve problems but might also create some communication issues that affect teamwork.
Good data leaders can fix this by:
- Creating a common language for the team: They should encourage everyone to use simple, clear words and avoid complex terms.
- Encouraging active listening and open talks: They should make a welcoming space where everyone can speak up about their ideas and worries.
- Using project management methods: They can apply tools and techniques for managing projects. This helps with clear task assignments, tracking progress, and communication among the team.
The Future of Data Teams: Trends and Predictions
The field of data science is always changing. New trends and technologies are impacting how data teams work. Organizations that accept these changes will be in a better place to use data’s powerful effects and stay ahead of their competitors. With the rising importance of AI and machine learning and a move towards more flexible structures, the future for data teams looks very promising. Gartner predicts that by 2024, 75% of organizations will adopt AI-powered augmented analytics to enhance decision-making processes. As we enter the AI era, business priorities and the means and methods for achieving them are swiftly changing. CIOs must adapt their strategies to develop teams capable of delivering business results.
The Growing Role of AI and Machine Learning in Data Analysis
Artificial intelligence (AI) and machine learning are not just ideas for the future. They are now important tools being used in data analysis. Data teams are using AI to automate tasks, make predictions more accurate, and find new patterns in big data.
For example, AI can now handle data cleaning and preparation. This helps data scientists spend their time on more complex work like building models and understanding results. By 2025, the global AI market is estimated to reach $190.61 billion, highlighting its growing significance in data analysis. The use of AI in data analysis will keep growing. It will help data teams get even more value from their data.
The Shift Towards More Agile Data Team Structures
Traditional, hierarchical organizational structures are giving way to more agile and adaptable data team structures. This shift is driven by the need for greater flexibility, faster iteration cycles, and improved responsiveness to ever-changing business needs. A survey found that 79% of organizations have been hindered by a lack of collaboration, emphasizing the shift towards more integrated and agile structures.
Agile Data Team Structure |
Characteristics |
Cross-functional Teams |
Data professionals from different disciplines work together on specific projects, fostering collaboration and faster decision-making. |
Self-managing Teams |
Teams have greater autonomy and ownership over their work, leading to increased accountability and motivation. |
Iterative Approach |
Projects are broken down into smaller, iterative cycles, allowing for continuous improvement and adaptation based on feedback. |
Anticipating the Next Big Thing in Data Technology
Data teams are always searching for new developments in data technology. They are eager to see how new trends will affect their work. One trend is edge computing. This is when data is processed and analyzed near its source. This helps reduce delays and allows for real-time insights. By 2025, IoT-generated data is estimated to reach 79.4 zettabytes, highlighting the growing importance of edge computing.
Also, quantum computing is developing quickly. It can process information much faster than regular computers. As data teams look at and use these new technologies, we will likely see more creative ways to use data that can change businesses for the better.
Case Studies: Successful Data Teams in Action
Looking at real examples of successful data teams gives us useful lessons on how different groups use data to get great results. Big companies in the Fortune 500 are creating cultures that focus on data. Some startups are even making their whole businesses based on strong data plans. These case studies show how much of a difference good, organized data teams can make.
How Fortune 500 Companies Leverage Data Teams for Innovation
Many Fortune 500 companies credit their success to using data. They rely on data to guide decisions at all levels. These businesses spend a lot to create strong data teams. They attract skilled people and provide them with the best technologies. A report indicates that 97% of Fortune 500 companies are investing in big data and AI to enhance their decision-making processes.
For example, a big retail company has used AI to create recommendation tools. By looking at a lot of customer data, their data team made a system that suggests products for each customer. This led to a big rise in sales and happier customers.
Startups That Have Built Their Success on Robust Data Strategies
Startups are known for being quick and creative. They often focus on their data strategy right from the beginning. They understand that data is very important for their growth. These companies use data to improve their products and services. They also study their target market better. This helps them make smart decisions that give them an advantage over their competitors. Statistics show that 90% of startups believe data analytics is crucial for their growth and competitive edge.
A great example is a ride-hailing startup. This company changed the transportation industry by using data analytics. Their data team created advanced algorithms that help find the best routes. They connect riders with drivers nearby and change prices based on real-time demand and traffic. This way of using data changed how people travel in cities and helped the startup become a leader worldwide.
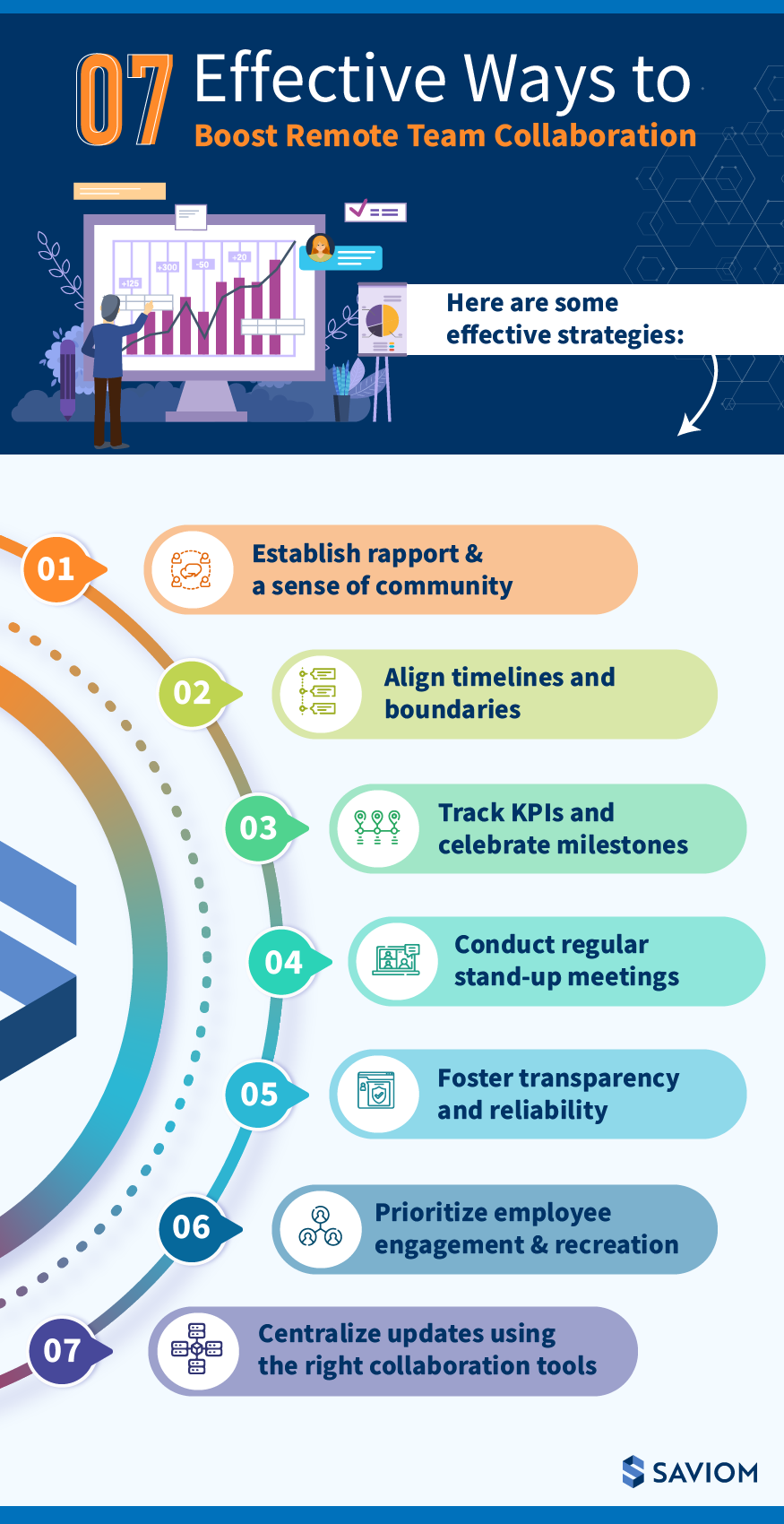
Dataforce’s Unique Approach to Empowering Data Teams
At Dataforce, we are very proud of how we help data teams. We know that to really use data well, organizations need the right people, the latest tools, and a strong support system. This helps them grow and do their best work.
We offer a wide range of services to meet the changing needs of data teams. These services include finding and training talent, as well as providing advice and help with putting strategies into action. Dataforce has successfully placed over 5,000 data professionals in various roles, demonstrating our expertise in strategic recruitment and talent placement.
Bridging Talent Gaps with Strategic Recruitment
Dataforce knows that finding the right people is key to building a strong data team. We are great at strategic recruitment. We link organizations to skilled data professionals who fit their needs well. Our experienced recruiters use a wide network and industry knowledge to find the best talent in data science, data engineering, data analysis, and other related areas. In 2023, Dataforce connected more than 1,200 candidates with leading employers, showcasing our commitment to bridging talent gaps.
We do more than just match resumes to job descriptions. Our careful screening process helps us find candidates with the right technical skills. We also look for strong problem-solving abilities, good communication skills, and a love for making decisions based on data.
Leveraging Salesforce Expertise for Data Team Efficiency
Dataforce is a Salesforce consulting partner. We know how to use Salesforce to help data teams work better. We focus on integrating Salesforce with other data sources. This gives a clear picture of customer data. With this, businesses can do better marketing, create more personalized experiences, and forecast sales accurately. Our Salesforce integration solutions have helped clients achieve a 30% increase in data processing efficiency.
Our team has certified Salesforce experts. They help companies set up, customize, and improve Salesforce solutions that fit their business needs. By using Salesforce, Dataforce helps data teams make their work easier, understand their data more deeply, and achieve great business results.
Partnering with Industry Giants for Mutual Growth
Dataforce knows that working together is important. We have teamed up with big names in data and technology. This helps us give our clients the latest ideas and top solutions. Dataforce has partnered with over 50 industry leaders, ensuring our clients benefit from cutting-edge innovations and best practices.
These partnerships help us stay updated on industry trends. This way, we can give our clients a better chance to succeed. We think that by collaborating, we can help each other grow, bring new ideas, and build a future that uses data better for businesses everywhere.
Conclusion
In conclusion, the different roles in data teams are very important for today’s businesses. Data scientists pull out valuable insights. Data engineers make sure data is easy to get and secure. Each role plays a big part. Working together, training, and solving challenges are vital for data team success. According to industry analysis, organizations with well-structured data teams are 3.5 times more likely to outperform their competitors. This includes balancing technical skills with business needs. As we move forward, using trends like AI and flexible setups will be essential. It is projected that by 2025, 90% of large organizations will have a Chief Data Officer, reflecting the growing importance of data teams in strategic decision-making. The future has exciting new changes for data teams. They will be crucial for smart decisions and growth in the digital world.
References:
- https://towardsdatascience.com/building-data-science-capabilities-means-playing-the-long-game-f68166ecf2f
- https://www.glassdoor.co.uk/Salaries/us-data-analyst-salary-SRCH_IL.0,2_IN1_KO3,15.htm
- https://www.gartner.com/
- https://medium.com/airbnb-engineering/at-airbnb-data-science-belongs-everywhere-917250c6beba
- https://www.gartner.com/en/articles/choose-adaptive-data-governance-over-one-size-fits-all-for-greater-flexibility
- https://aws.amazon.com/data/cdo-report/
- https://devskiller.com/it-skills-report/
- https://medium.com/@kozyrkov
- https://github.com/microsoftdocs/architecture-center/blob/main/docs/data-science-process/roles-tasks.md
- https://project-management.com/6-tips-to-plan-and-manage-a-successful-bi-project/