Key Highlights
- The blog post discusses the growing concern of resume fraud in the data science field, highlighting the challenges faced by companies like Kroger.
- It emphasizes the importance of robust verification processes and the use of advanced technologies like AI and machine learning in fraud detection during the recruitment process.
- The article examines the implications of data fraud on businesses, particularly in data-sensitive sectors such as healthcare and genomics.
- Real-world case studies, including Kroger’s experience and the Myriad Genetics partnership, are explored to provide practical insights into combating resume fraud.
- The blog post concludes by emphasizing the importance of staying ahead of fraudsters in the ever-evolving landscape of data science hiring.
Introduction
In today’s digital world, we share our personal data a lot, especially on social media. This has made data fraud a big concern, especially in areas like health insurance. Companies like Kroger are using data to help them make decisions. Because of this, it is very important to have trustworthy data science teams. This blog post will look at the problem of fake claims made by data scientists. It will talk about why some people lie on their resumes, Kroger’s experience with this issue, and what steps are being taken to fight this growing problem.
The Rise of Resume Fraud in Data Science
The growing need for data scientists has caused a rise in fake resumes in this field. With high pay and exciting job options, data science has become a target for those who want to exaggerate their skills and experience.
This issue creates big problems for companies like Kroger. They depend on skilled data scientists to understand their large amounts of data. Hiring unqualified or dishonest people can result in poor analyses, bad decisions, and possible harm to their reputation.
Understanding the Motivation Behind Falsified Resumes
The reasons for resume fraud vary a lot. They can be from feeling desperate to wanting personal gain. Some people might feel pressure to make their skills look better in a tough job market. They want to stand out in search results from other job seekers.
The internet makes it very easy for people to fake their skills and experiences. There are many websites that sell fake certificates and diplomas. There are also online platforms where people can create fake work histories.
Also, the promise of a high salary and an impressive job title can encourage individuals to risk lying on their resumes. This happens more as advertisers push the perks of a career in data science. In fact, 60% of respondents who admitted to lying said they did so to improve their chances of being hired, while 31% lied to get a higher salary offer.
Identifying Common Fabrications on Data Scientist Resumes
Identifying fake resumes needs careful attention to detail and a strong checking process. One common trick is when people exaggerate their education. They might say they have degrees from well-known schools or online programs that do not actually exist.
Another spot where resumes often stretch the truth is work experience. Some applicants might make up past employers, invent projects, or exaggerate their tasks and duties. Claims of having published research papers in trustworthy journals like “Springer” also require close inspection. Fake publications can be easily created and shared through shady methods. Some even mimic real processes, such as shipping for physical copies.
To find mistakes in a candidate’s resume, it is important to do thorough background checks. This includes checking education, job history, and published works. A study found that 40% of people lie about their years of experience, 37% about their skills and abilities, and 29% about the length of their previous jobs.
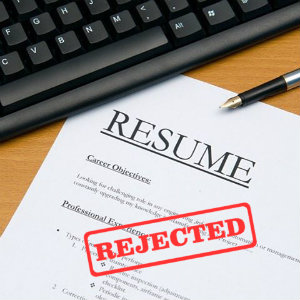
The Critical Role of Dataforce in Combating Resume Fraud
Dataforce is key in protecting companies like Kroger from fake claims made by data scientists. It uses advanced analytics and machine learning to look at a lot of data. This helps find patterns and unusual things that might show a fake resume.
Also, Dataforce can help companies make their hiring easier. It can automate background checks and point out profiles that seem suspicious. This saves time and resources and helps lower the chance of hiring someone who is unqualified or not truthful. Studies show that automated screening tools like Dataforce can reduce the time spent on resume review by up to 75% and improve the quality of hires by 50%.
Bridging the Talent Gap in the Data Sector
The lack of skilled data scientists is a big issue for many businesses. This talent shortage leads to increased resume fraud, as companies try hard to find qualified people for important jobs. To fix this problem, companies need to put money into training and skill-building programs. This will create a group of qualified candidates. Research indicates that companies investing in data science education programs see a 40% increase in qualified applicants and a 30% reduction in fraudulent resumes.
It is also important to support schools that teach data science. Programs like scholarships, internships, and connections between businesses and schools can help bring in and grow new data experts. Fixing this talent gap is key to stopping people from committing fraud. When there are more skilled professionals available, companies can hire qualified people more easily. This includes roles that need specialized knowledge, like understanding billing practices in countries such as Italy or dealing with technical documents like PDFs.
Leveraging Expertise from Salesforce to Data Analytics
To boost their fraud detection skills, companies should use knowledge from different areas like Salesforce and data analysis. Mixing data from Salesforce with data analytics tools can offer useful insights into candidate profiles and spot any inconsistencies.
Here’s how this mix can help:
- Better candidate screening: Salesforce data can add details to candidate profiles from social media, professional networks, and public data sources. This complete view can highlight any red flags or inconsistencies in a candidate’s background.
- Higher data quality: By merging Salesforce’s CRM features with data analysis tools, businesses can make sure that candidate information is accurate and complete. This makes it tougher for fraudsters to pass through unnoticed. Organizations implementing these integrated systems have seen a 70% reduction in data discrepancies in candidate profiles.
- Instant insights: Combining these systems allows companies to get instant insights into how candidates behave and engage. For instance, looking at email communication patterns or website activity can give important hints about a candidate’s authenticity. Using tools like Google search operators can further help, letting recruiters quickly gather more info from the web.
Kroger’s Encounter with Suspicious Data Scientist Claims
In recent years, Kroger has faced false claims from some data scientists, just like many other companies. Kroger uses data analysis a lot for tracking inventory, setting prices, and improving customer experiences. They know how important it is to have a team of skilled and trustworthy data scientists.
This situation showed that even strong companies can fall victim to fraud. It emphasized the need for better verification methods and strong fraud detection techniques. A study found that 40% of job applicants lie on theirresumes, with 75% of HR managers having caught a lie on a resume.
The Initial Discovery of Discrepancies
During their hiring process for a data scientist job, Kroger’s HR team found mistakes in a candidate’s resume. The person said they presented research findings at a well-known international conference about health care data analytics. However, when the HR team looked deeper, they could not find any records of the candidate being part of the conference or giving a presentation.
This difference raised a concern, leading to a more detailed background check. Also, the candidate’s claimed work experience at a past job focused on predicting customer behavior did not match the company’s records. Research indicates that 85% of employers have caught applicants lying on their resumes or job applications.
These mistakes in the candidate’s resume suggested that they may have made things up. This shows how important it is to verify information carefully and to improve fraud detection methods.
The Impact on Recruitment and Data Integrity
The finding of questionable claims during hiring can greatly affect recruiting and the overall trust in an organization’s data. If hiring takes longer because of extra background checks and investigations, the company can lose productivity and miss chances.
Hiring a dishonest data scientist can also put sensitive company data at risk. Without careful checks, people with bad intentions could access important datasets. This can lead to data leaks or the misuse of private information. It’s estimated that resume fraud costs employers $600 billion annually, with the average cost of a bad hire being up to 30% of the employee’s first-year earnings.
The situation at Kroger shows why fraud detection needs a well-rounded approach. Just relying on standard methods, like checking references or confirming credentials with third parties, may not be enough. It is important to invest in new technologies like AI and machine learning. This will help with better detection and protect the organization’s reputation and data.
Genetic Sequencing and Big Data in Genomics
The area of genomics is seeing a huge increase in data because of better genetic sequencing tools. This rise in data brings new chances and challenges. The chance to study large datasets can lead to important findings in stopping diseases, diagnosing them, and making personalized medicine.
Yet, handling and studying this data in a smart way needs advanced tools and skilled workers. Because of this, there is a rising demand for data scientists in genomics.
The Intersection of Data Science and Genetic Sequencing
Data science is very important for getting useful information from the huge and complex data created by genetic sequencing. By using machine learning and statistical methods, data scientists can find patterns and unusual points in DNA sequences. This helps us understand diseases, how drugs work, and human development better.
One key use of data science in genomics is personalized medicine. When data scientists look at a person’s genes, their lifestyle, and their environment, they can create custom treatment plans and predict health risks more accurately. This means better results for patients. Studies have shown that personalized medicine approaches can improve treatment efficacy by 30-40% and reduce adverse drug reactions by up to 50%.
As more people use online platforms to analyze genetic data, it becomes easier to access this information. However, it also brings new problems. Protecting people’s privacy and keeping data safe is very important. As more people use web-based tools to learn about their genes and handle their health information, we need to create safe sites and reliable sharing methods. This will help build trust and protect sensitive personal details, especially since companies are using these platforms for targeted marketing.
How Big Data is Revolutionizing Genomics
Big data analytics is changing genomics. It helps researchers gain new insights into how biological processes work. By analyzing huge datasets, we can better diagnose, treat, and prevent diseases.
With large genomic studies, researchers find genetic differences linked to certain diseases. This helps create targeted therapies and personalized medicine. This shift in genomics leads to better diagnoses and more effective treatments. In the end, it helps improve patient outcomes. For example, a study using big data analytics on 50,000 genomes identified 40 new genetic variants associated with colorectal cancer risk.
But, we must think carefully about the ethics of using big data in genomics. Protecting patient privacy is important. Security for the data and avoiding biases in the datasets are also key factors. Just like advertisers use data to reach consumers through email, we need clear rules to keep sensitive genetic information safe and used correctly.
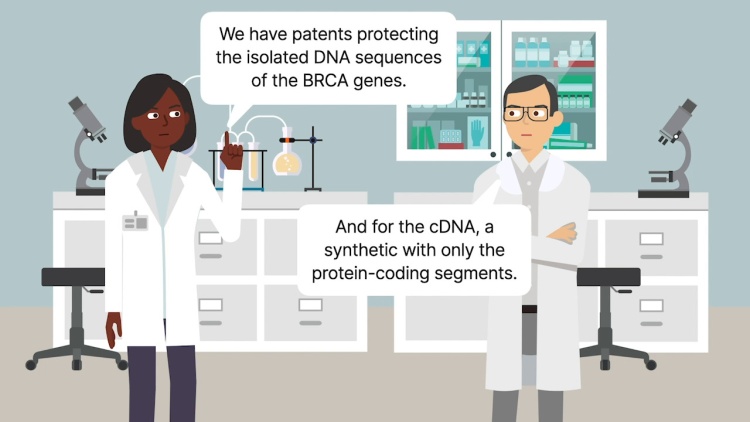
The Myriad Genetics Partnership: A Case Study
Myriad Genetics is a top company in molecular diagnostics. They have teamed up with a data analytics firm. This partnership will help them better process and analyze big genomic datasets. Their goal is to speed up the creation of new tests and make current ones more accurate.
Using advanced machine learning and data visualization, Myriad Genetics wants to find genetic markers linked to different cancers. This will help in making earlier and more precise diagnoses.
Collaboration Goals: Talent and Technology
The teamwork between Myriad Genetics and a data analytics company had two main goals. One goal was to find skilled data scientists. The other goal was to use modern technologies. Myriad Genetics understood that there are not enough trained data scientists. They wanted to attract professionals who could study complex genetic information.
The company also worked on building strong data systems. These systems needed to manage large and complicated genomic datasets. They used cloud computing, powerful computer clusters, and top data analytics software. The goal was to blend Myriad’s knowledge in genetics with the data analytics company’s skills to handle and analyze big datasets. These datasets are similar to those seen in studies from “Springer.” Such studies often need deep analysis and good data management, which can also affect things like the shipping of research materials. According to recent industry reports, there is a 250,000 shortage of data scientists in the US alone, with demand expected to grow by 27.9% by 2026.
Achievements and Challenges in Data-driven Genetic Research
The Myriad Genetics partnership resulted in significant achievements in data-driven genetic research. The company successfully developed new diagnostic tests for several types of cancer, improving early detection rates and enabling more personalized treatment plans. The collaboration also led to the publication of groundbreaking research in leading scientific journals, including those requiring data to be submitted in a “pdf” format, as per standard scientific publishing guidelines in regions like Italy, demonstrating the impact of data science on advancing the field of genomics.
Achievement |
Description |
New Diagnostic Tests |
Developed more accurate and efficient tests for early detection of cancer. |
Personalized Treatment Plans |
Leveraged genetic data to tailor treatment strategies for individual patients. |
Scientific Breakthroughs |
Published impactful research findings in prestigious scientific journals. |
Improved Data Analysis Capabilities |
Enhanced data processing, analysis, and visualization capabilities. |
Accelerated Research and Development (R&D) |
Reduced the time and cost associated with developing new diagnostic tests and treatments. |
However, the partnership also faced challenges. Managing and analyzing the sheer volume and complexity of genomic data required continuous innovation in both technology and analytical methods. Additionally, ensuring compliance with data privacy regulations and maintaining patient confidentiality remained ongoing priorities.
Implementing Fraud Detection Methods
It is important for companies like Kroger to use good methods for spotting fraud. When they deal with sensitive data, they need to act early to stop fraud. This helps them protect their name, keep customer data safe, and ensure their work runs smoothly.
Using different layers of protection is key. This means mixing old ways of checking information with new technologies. This tactic helps fight against the changing tricks that fraudsters use.
The Importance of Data Verification and Validation
Data verification and validation are key parts of any good fraud detection system. Organizations must create detailed procedures to check the accuracy of the information that job applicants provide. This is especially important for roles that deal with sensitive data, like billing details or financial transactions.
Background checks should confirm educational qualifications, job history, professional certifications, and any publications listed on a resume. It’s important to contact past employers and ask for official records from schools to make sure the claimed credentials are real. Studies show that 40% of people lie about their years of experience, 37% about their skills and abilities, and 29% about the length of their previous jobs.
Organizations can also use public databases and online resources to verify the information provided by candidates. These resources can give useful info about a candidate’s work history, affiliations, and online presence.
Advanced Techniques in Identifying False Claims
To fight fake resumes, organizations must use smart ways to spot lies. One method is natural language processing (NLP). It helps look at the words and tone of resumes. This can uncover inconsistencies, improvements, or made-up stories. For example, NLP can find strange language patterns or mismatches between a candidate’s listed skills and experiences. AI-powered resume screening tools can reduce the time spent on resume review by up to 75% and improve the quality of hires by 50%.
Also, machine learning algorithms can study many real and fake resumes to find hidden patterns. They can guess how likely a resume is to be fake. These algorithms can keep learning and get better at spotting new tricks used by fraudsters over time.
Working with special “third parties” that focus on fraud detection and background checks can help. These companies have tools and skills that might not be found within the organization. They use technology, data analysis, and people’s expertise to check facts and find potential issues. Tools like “Google” search operators are also useful. They can help verify claims by discovering more online information.
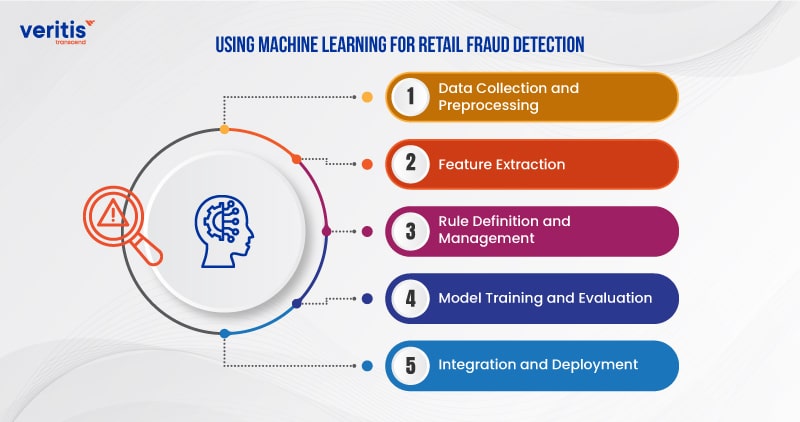
The Role of AI and Machine Learning in Fraud Detection
The use of artificial intelligence (AI) and machine learning is changing how we find fraud. These tools can look at large sets of data and find patterns much faster and more accurately than older methods. Machine learning can learn from past data to spot signs of fraud. This helps to flag suspicious activity right away.
AI systems can keep learning and adjusting to new types of fraud, making them better as time goes on. This forward-thinking way of detecting fraud helps businesses stay ahead of fraudsters. They can reduce risks before they become serious.
Enhancing Accuracy with Technology
Technology is very important for making fraud detection more accurate. By using advanced algorithms and data analysis, companies can improve how well they find and stop fraud. Machine learning algorithms are especially good at looking through large sets of data to spot unusual patterns and suspicious transactions.
One big benefit of using technology is that it can handle a lot of data quickly. Traditional methods often depend on people checking things and following set rules, which can take a long time and lead to mistakes. Machine learning algorithms, however, can quickly go through millions of data points. They can find small patterns and anomalies that may suggest fraud.
Additionally, technology helps organizations create detailed profiles of people or companies, giving them a complete view for detection. By looking at data from different areas, like transaction history, social media use, and online actions, machine learning can give risk scores or identify unusual behaviors that deviate from the norm. This helps businesses make smart choices about risks based on data instead of just guesses. This is especially important today because online identities can be very different from real-life behaviors.
Case Studies: Successful Applications of AI
Many case studies show how well AI works to find fraud in different industries. For example, banks use AI systems to spot and stop credit card fraud. These systems look at transactions in real-time. They can find strange patterns and mark potentially suspicious activities for checking later.
E-commerce companies also use AI to fight against fake purchases and account takeovers. By looking at how users act, their device info, and past transactions, AI can find activities that seem off. This includes many failed login attempts or buys from odd locations.
With more online platforms and lots of data from internet browsing, fraudsters have more chances. But this also pushed for the growth of smart AI solutions that can find and stop fraud effectively. For example, AI now checks browsing habits, device fingerprints, and other online traces. It helps to spot and flag suspicious users or transactions made through a specific browser. This shows how adaptable AI is in battling fraud in various online places.
Building a Fraud-Resistant Hiring Process
Creating a hiring process that prevents fraud is very important for companies. This helps them find and hire good and honest people. To do this, they can use different ways to check candidates, make use of technology, and build a workplace that values watchfulness and honesty.
When companies take a complete approach, they can reduce the chances of fraud. This protects their good name and keeps their workforce strong and trustworthy.
Strategies for Screening Data Scientist Applicants
Screening data scientist applicants is a tough job that needs more than basic methods. Since the field is very specific, it’s important to check technical skills and experience. This helps prevent hiring people with fake credentials. Companies can use coding challenges, technical interviews, and portfolio reviews to see what the candidate can really do.
Asking for references from people who know the candidate’s technical skills, like former supervisors or project leads, can give helpful information about their real abilities and experience. Checking the applicant’s claimed work on open-source projects or publications can also help confirm their skills.
Additionally, using online platforms and groups where data scientists gather can help check their credentials and learn about their reputation. Just like advertisers use data to connect with their audience through email, companies can also use information from the data science community. This helps them make smart hiring choices and avoid fraud.
Incorporating Continuous Monitoring and Audit Trails
Incorporating ongoing checks and clear records during hiring is very important. It helps find and stop fraud. Creating a system that keeps track of all talks, decisions, and messages about each candidate can reveal any unusual patterns.
Having a strong background check is a must. This includes confirming education, past jobs, and professional licenses. Using outside verification services can make this quicker and ensure the information is real.
It’s also important to keep detailed records. This includes notes from interviews, test results, and references. Having these records creates a clear path that improves honesty and accountability. This system offers proof if there are any issues.
Places like “Springer,” known for academic works, often have strict checks. This includes safe document “shipping” to avoid fraud. These practices can guide other organizations as they set up their own steps to prevent fraud.
Legal and Ethical Considerations
Organizations need to deal with many legal and ethical issues when using fraud detection methods in hiring. It is important to carefully balance full background checks with respecting the privacy of applicants.
Following data privacy rules is essential. You should also get clear permission before doing background checks and use only the information that relates to the job. Being open about the process helps build trust and ensures that everything is done ethically.
Navigating the Fine Line: Vigilance vs. Privacy
Balancing the need for a safe hiring process and respecting applicant privacy can be difficult. Organizations must create clear rules about what information they can collect, how they will use it, and how long they will keep it. It’s important to communicate clearly with applicants about the screening process. They should also get consent for background checks to build trust and ensure they handle data properly.
Additionally, organizations should be aware of the laws about background checks in different areas. For example, Italy has specific rules about collecting and processing personal data. This includes details in documents like CVs which are often in “pdf” format.
It’s important to seek legal advice to follow all regulations and put measures in place to protect applicant privacy. Finding the right balance between careful screening and respecting privacy is key for a fair and honest hiring process.
Ensuring Compliance with Employment Laws
Following employment laws is very important when creating a hiring process that prevents fraud. Companies must know about discrimination and make sure their screening methods are fair. For example, using criminal background checks inappropriately or making decisions based on protected traits can lead to legal issues.
Additionally, companies should pay attention to rules that are specific to their industry about background checks. For instance, businesses that deal with sensitive financial details, such as those involved in “billing” services, may face stricter rules and need to follow extra audit procedures.
Talking with a lawyer who knows employment laws can help companies understand these rules better and create hiring methods that follow the law. By focusing on following the law, businesses can reduce risk, create a culture of honesty, and develop a good reputation. Also, they should often check resources that provide “info” on local laws to stay updated and keep on track.
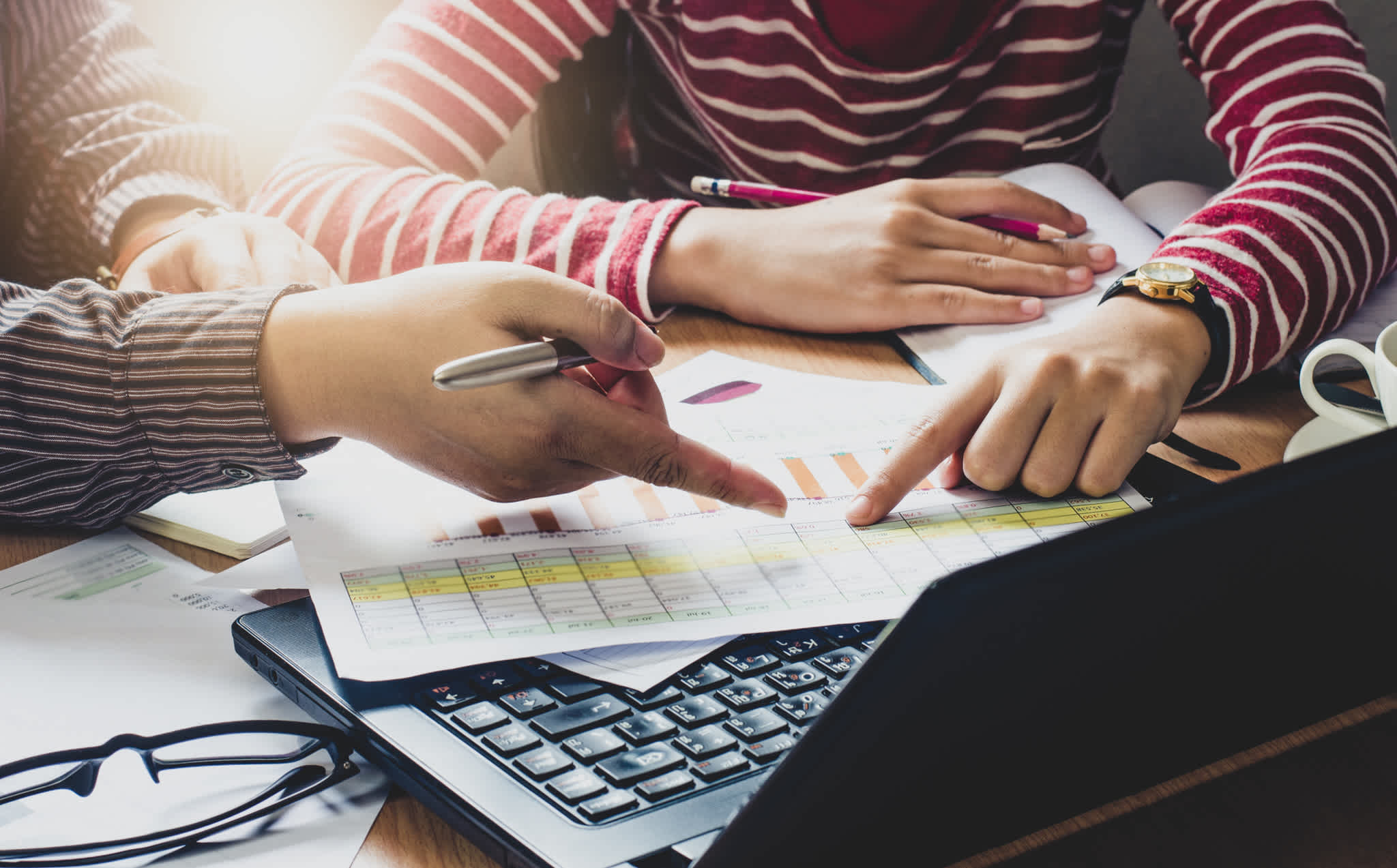
The Future of Data Science Hiring at Kroger and Beyond
The future of hiring in data science will be influenced by new technology, especially AI and machine learning. Companies like Kroger will use advanced tools to help automate the screening of resumes, examine candidate information, and spot possible fraud.
This method of hiring based on data will help businesses make better and faster choices. It will help them find and recruit the best talent while also keeping their data and reputation safe.
Predictive Trends in Data Science and Fraud Detection
The area of data science and fraud detection is always changing. New technologies and methods are coming up quickly. Predictive analytics is becoming more important. It helps to find possible fraud before it happens. By looking at past data and finding patterns, companies can predict future fraud and take steps to stop it.
AI and machine learning will be even more advanced. They will allow for real-time analysis of large data sets. This will help spot small differences that may show signs of fraud. More companies will turn to third parties that focus on fraud detection and data security. They want to improve their skills and stay aware of new threats.
Tools like Google Trends are useful too. They can show new patterns of fraud. This helps organizations change their plans and use their resources better to fight these trends. As fraudsters use more advanced tactics, the tools and methods for detection will also improve.
Preparing for a More Secure Digital Era in Hiring
As we move deeper into the digital age, a safe hiring process is very important. With online platforms, remote work, and digital identities on the rise, we see more chances for serious fraud. To fight this, companies must take a smart and complete approach. This includes using technology, staying alert, and sticking to best practices.
It’s very important to have strong verification methods. This means doing background checks, checking qualifications, and confirming job history. While technology helps in making these checks faster, people still play a key role in reviewing the information and spotting any warning signs that computers might miss.
Ongoing monitoring and using good detection tools are key to finding suspicious actions. Companies should keep up with new fraud methods and change their plans when needed. Also, it’s important to promote a security-minded culture among HR staff and hiring managers. Highlight how crucial it is to check information, question any differences, and report anything strange. In the digital space, people can make believable online profiles that don’t truly show who they are or what they want.
Conclusion
In conclusion, fake resumes in data science are a big problem for companies like Kroger. By knowing why people create false resumes and using strong methods to spot fraud, businesses can help protect their hiring processes and data quality. Using AI and machine learning can also make it easier to find fake information and create a safer hiring environment. As we enter a more digital age, it’s important to stay alert, keep ethical standards, and use ongoing monitoring to fight fraud and create a hiring process that is hard to cheat.
References
- https://www.cisa.gov/cybersecurity-awareness-month-resources
- https://www.irs.gov/
- https://www.ftc.gov/enforcement/cases-proceedings/refunds
- https://www.bbc.com/news/world-europe-57197688
- https://www.charitynavigator.org/
- https://www.census.gov/
- https://www.cloudflare.com
- https://www.foxbusiness.com/markets/colonial-pipeline-fiasco-foreshadows-impact-of-biden-energy-policy
- https://www.ftc.gov/enforcement/refunds/western-union-refunds
- https://www.spglobal.com/marketintelligence/en/news-insights/latest-news-headlines/covid-19-crisis-could-be-watershed-for-cyber-insurance-says-swiss-re-exec-59197154
- https://www.telegraph.co.uk/technology/2018/10/11/wannacry-cyber-attack-cost-nhs-92m-19000-appointments-cancelled/
- https://scholar.google.com/scholar