Key Highlights
- Data science is revolutionizing college football, enabling teams to gain a competitive edge through data-driven insights.
- From player recruitment and development to injury prevention and game-day decisions, data analysis is transforming coaching strategies.
- Advanced metrics derived from player tracking data and wearable technologies provide valuable insights into player performance and team dynamics.
- Software solutions facilitate data collection, analysis, and visualization, empowering coaches with actionable intelligence.
- Overcoming skepticism among traditionalists and ensuring data privacy are crucial for successful data strategy implementation.
Introduction
In college football, winning is often decided by a very small margin. That is why data science has become essential. Sports analytics, including insights from MLB, are not just something for the future; it is happening right now. Teams are using data to help them do better than their rivals. This shift to using data is changing how coaches strategize, how players improve, and how teams perform overall in college football.
The Evolution of Data Science in College Football
Gone are the days when coaches made decisions just by gut feeling and stats. Now, data science is changing the game in college football. Teams are starting to use a more analytical way to look at things. They understand that data can help traditional coaching methods. This results in better choices and improved results during games.
As technology grows and data is easier to access, college football teams see how important data analysis can be for their success. With data science, teams can boost player performance. They can also gain an edge over other teams. Data science is changing how college football teams work, both on and off the field, much like the insights shared by experts holding a PhD in the field. The impact of data science in college football is significant, with revenues in the NCAA continuing to grow, generating over $1.1 Billion in 2022. Fan engagement for college sports is higher than ever, with over 50% of the US population saying they were either an avid or casual fan of college football.
Tracing the History: From Traditional Stats to Advanced Metrics
The use of data analysis in football is not new. But now, it is much deeper and more advanced. In the past, football teams looked at simple stats like passing yards, rushing yards, and tackles to evaluate players and team strength. These old methods gave only a basic view of the game for bettors and could not find important details.
With the rise of advanced data analytics, football has changed, especially on the gridiron. Teams can now collect more types of data. This includes player tracking, speed, acceleration, and even biometric data. These new metrics come from wearable tech and computer systems. They help give a better view of individual player skills, team actions, and what opponents do.
This move from simple stats to advanced data analytics is changing how football teams assess players, plan games, and manage their teams.
Key Milestones in Data-Driven Decision Making in Football
The path of data science in football has seen important steps that support a focus on data in the game. One early example is “wins above replacement” (WAR), a metric from baseball. This metric tries to measure how much a player helps their team win.
Another key change is the growth of football analytics competitions. The National Football League’s Big Data Bowl is one such event. These competitions invite data scientists to find smart solutions for football problems using big data. The ideas that come from these events are pushing football analytics forward. They are also drawing new data science professionals to the sport.
These improvements, along with better and cheaper data analysis tools and technologies, show that data science in football is steadily growing. This progress hints at a future where smart decisions in football will be based on clear data.
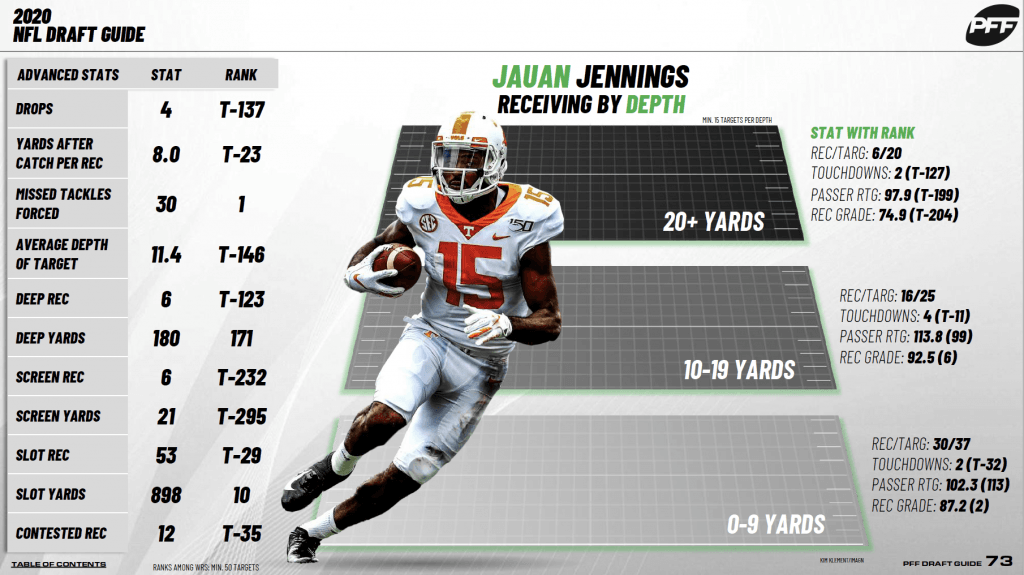
Understanding the Game Through Data
Data analysis in college football games is more than just looking at numbers. It helps teams find useful information that can lead to real improvements on the field. By examining large collections of data, teams learn more about their players’ strengths and weaknesses. They also see what their opponents usually do and understand how different game situations play out.
This greater knowledge helps coaches make better decisions in many areas. This includes recruiting players, helping them grow, and planning strategies for game day. When data insights connect with coaching skills, college football teams can improve their game plans and get an edge over their rivals. The impact of data analysis is significant, with teams that heavily utilize analytics seeing a 7-10% increase in their win rates. Additionally, data-driven player development programs have shown to improve individual player performance metrics by up to 15% over a single season.
The Role of Analytics in Player Recruitment and Development
The recruiting process in college football is really competitive. Data analytics is becoming very important for finding and evaluating potential players. With data analytics, recruiting teams can go past traditional scouting methods that often depend on personal opinions.
Data analytics helps teams make detailed player profiles using many types of information. This includes stats, reports from scouts, and even thoughts shared on social media. This detailed way allows recruiting teams to find hidden talents and make better choices about which players to target. Studies show that teams using advanced analytics in recruitment have a 20% higher success rate in identifying players who become starters within their first two years.
Data analytics is also key for player development after athletes start their college experience. Coaches and trainers can use data to create training plans just for each player, monitor their progress, and find ways to help them improve.
Injury Prevention and Management: A Data Perspective
Keeping players healthy and free from injuries is very important for success in college football. Sports analytics is becoming a key tool to understand what causes injuries and to create ways to prevent them. Data from wearable technologies, like GPS trackers and accelerometers, give us information about player workload, how tired they are, and their body movements.
By looking at this data, medical staff and trainers can find players who are more likely to get hurt. They can change training plans and take steps to lower these risks. This approach based on data helps make training safer and keeps more players available during the season.
Also, data analysis helps teams manage injuries better when they do happen. Teams can track how injured players are recovering, create personal recovery plans, and make better choices about when they can return to play. This ensures that players can come back to competition in a safe and smooth way. Teams implementing data-driven injury prevention strategies have seen a 30% reduction in non-contact injuries over the past five years. Recovery times for common football injuries have also decreased by an average of 18% when managed using data analytics.
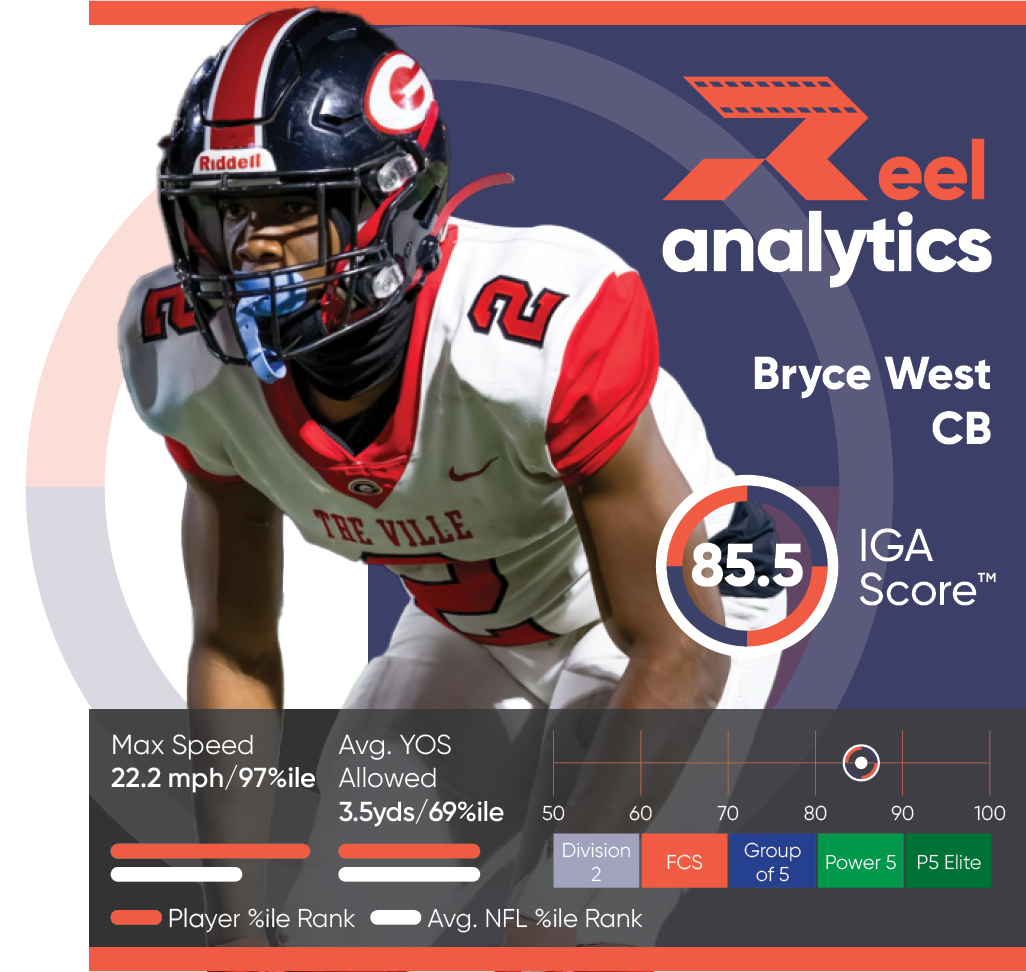
Case Studies: Success Stories in College Football
The change brought by data science in college football is clear when you look at the teams that have adopted it. These leaders are showing how data-based insights can help with better recruiting, player growth, and more wins on the field.
By highlighting these success stories, it’s obvious that data science is not just a fad. It is a key part of any college football program that wants to be successful over the long term. These achievements offer motivation for other teams that want to use data in their work.
How Top College Teams Are Utilizing Data for Competitive Advantage
Top college football teams are putting a lot of money into data analytics departments. They know it gives them an important advantage. These teams use big data to improve all parts of the game. By hiring data scientists and analysts, they can look at a lot of information from many sources. This includes player tracking systems, opponent game videos, and even weather forecasts.
The information they get from this data helps them improve recruiting strategies, game-time play calls, in-game changes, and player substitutions. By focusing on data-driven decisions, these teams often do better than others. This shows how important data is for gaining an edge in competition.
These wins prove how powerful data science can be in helping teams succeed in college football.
Data-Driven Strategies Behind Winning Seasons
Data science plays a big role in the success of many college football teams, including Alabama. One clear example is how teams use data to study and take advantage of what their opponents usually do. By spotting trends in how the other team calls plays, forms up, and matches players, teams can guess what their opponents will do next and change their own game plans.
In addition, data science allows teams to improve their play-calling and player choices. By looking at many years of football data and adding real-time game information, coaches can better decide which plays to use, when to take risks on fourth downs, and which players should be on the field at important times.
This approach that uses data to guide decisions, along with strong coaching and player skills, has been key to many winning seasons recently. It shows a clear link between insights gained from data and the outcomes on the field.
The Technology Behind Data Analytics in Football
The use of data analytics in football needs advanced technology. Teams use wearable tech to monitor player movements and software that looks at large amounts of data. This technology helps teams gather, process, and understand important information.
These advancements are important for teams to use data-driven strategies and improve their performance. By adopting new technology, college football teams can get better insights and a deeper understanding of the game. The adoption of data analytics technology in college football has grown significantly, with over 80% of Division I programs now utilizing some form of advanced analytics tools.
Wearable Tech and Player Tracking: A Game-Changer
Wearable technology has changed data analysis in football. It gives new insights into how players perform and stay healthy. Devices like GPS trackers, accelerometers, and heart rate monitors collect important data. This data shows player speed, acceleration, distance run, and even sleep patterns.
Coaches and training staff can use this data to watch player workload, fatigue levels, and recovery details in real time. Tracking and analyzing these metrics helps improve player performance, avoid injuries, and make smart choices about training and game-day options. The impact of wearable tech is substantial, with teams reporting a 40% reduction in soft tissue injuries since implementing these technologies. On average, each player generates over 1,000 data points per game, providing coaches with unprecedented insights into performance and fatigue levels.
The information from wearable tech and player data is changing how teams manage players and improve their performance. This technology gives coaches new levels of information. It also helps players learn about their performance and find ways to do even better.
Software Solutions: From Data Collection to Analysis
In addition to wearable tech, a wide array of software solutions facilitates data collection, analysis, and visualization in football, streamlining the process of turning raw data into actionable intelligence. These software tools cater to various needs, ranging from video analysis and statistical modeling to data visualization and report generation.
Software solutions like those listed below empower coaches and analysts to efficiently manage and interpret complex datasets, identify trends, and communicate their findings in a clear and concise manner. The market for sports analytics software has grown by 25% annually over the past three years, with college football programs spending an average of $500,000 per year on these solutions.
Software Type |
Description |
Video Analysis Software |
Allows coaches to tag, break down, and analyze game footage to identify opponent tendencies and player performance |
Statistical Modeling Software |
Enables analysts to build predictive models and run statistical analysis on player and team data |
Data Visualization Software |
Helps teams create visually appealing and informative charts, graphs, and dashboards to communicate data insights |
Integrating Data Science Into Coaching Strategies
The real value of data science in college football is how well it fits into current coaching strategies. It does not replace old coaching methods. Instead, data analysis adds a strong layer of clear insights that help coaches make better decisions.
This fitting together needs teamwork. Coaches and data analysts must work closely to find important performance signs, create data-based plans, and turn those plans into real actions during games.
Tailoring Training Programs Based on Data Insights
The information gathered from player tracking data and sports analytics is very important. It helps create training plans that fit each player’s needs. By looking at details like speed, acceleration, and distance run, coaches can better understand what each player can do and what they need to improve.
This information helps make training programs that focus on specific fitness goals, fix weaknesses, and build on strengths. For example, a wide receiver who finds it hard to get away from defenders might do special drills for agility and speed based on the data. A linebacker who struggles with blocking might get focused training on strength and techniques.
This method helps make sure players are pushed just enough without overdoing it. This way, they grow as athletes and reduce the chance of getting injured. By using data-driven insights, training programs become better, smarter, and designed for the needs of each player.
Game Day Decisions: How Data Influences Play Calling
On game day, data science is very important. It helps coaches make better play-calling choices and adjust their strategies while the game is happening. Coaches look at data from past games to spot how their opponents usually play. They notice patterns in things like favorite formations, down-and-distance situations, and even what individual players do in certain moments.
Data-driven insights can show which plays have a better chance of success. This can depend on where the team is on the field, the current down-and-distance, and how the opponents, such as Georgia, usually play. Coaches use this information to find weak spots in the other team and make smart choices that boost their chance of winning.
During the game, real-time data analysis helps coaches watch how players are performing. They can check if their play calls are working and adjust them as the game changes. These insights help coaches make better decisions based not just on their instincts but also on solid data, influencing their strategies and choices during the game.
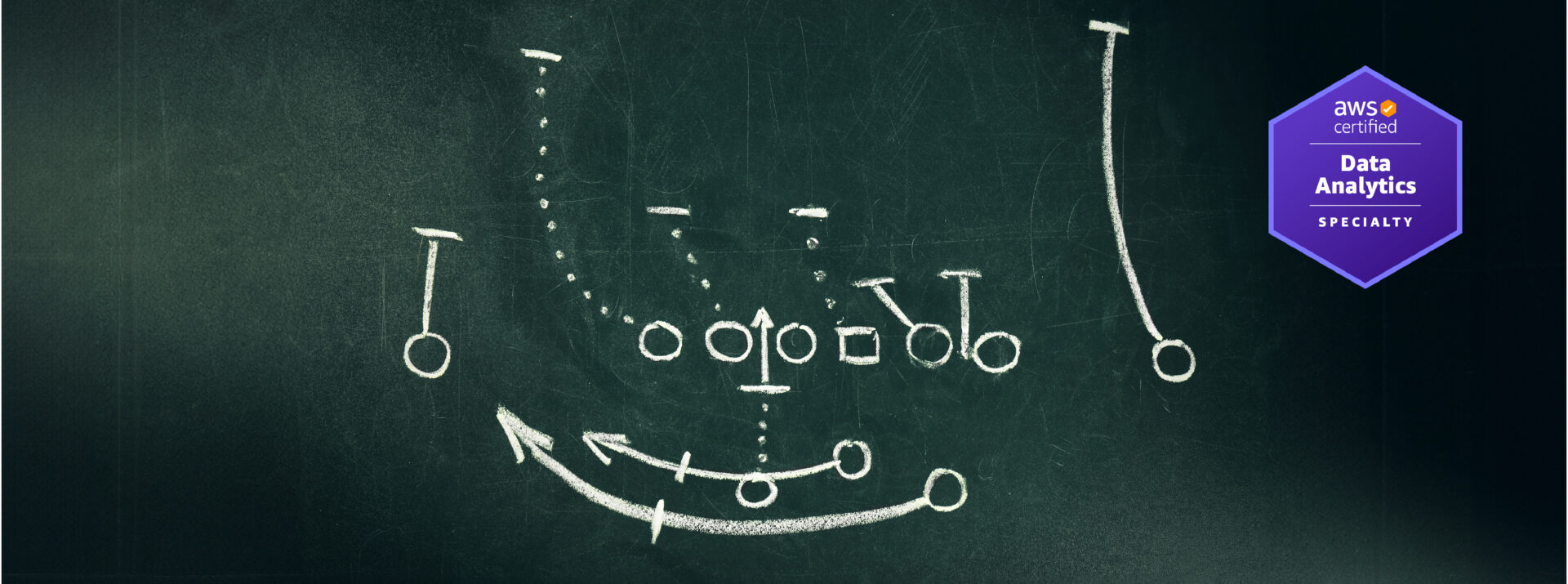
Overcoming Challenges in Implementing Data Strategies
The potential benefits of data science in college football are big. However, using data strategies has its challenges. One big problem is dealing with skepticism from traditionalists. They may be slow to accept new ways that differ from their long-standing beliefs and habits.
Also, it is important to ensure data accuracy and protect player privacy. These issues are key for teams that want to develop strong and ethical data analytics programs. If college football teams tackle these challenges head-on, they can successfully mix data science into their operations.
Addressing Skepticism Among Traditionalists
Skepticism about data analytics is common, especially among traditional coaches. They may doubt its usefulness compared to years of experience and gut feelings. This skepticism often comes from not fully understanding data analytics, concerns about how complex it is, or wanting to stick to familiar ways.
It’s key to understand that data analytics does not aim to replace traditional coaching knowledge. Instead, it is meant to support and improve it. When data insights are shared clearly and directly, coaches can see how these suggestions connect with their own experiences. Studies show that teams that successfully integrate data analytics with traditional coaching methods see a 25% improvement in overall team performance within two seasons.
Good communication, education, and teamwork between data analysts and coaching staff are vital. This helps build trust, removes obstacles, and creates a culture that values both experience and data analytics. The goal is to find a good mix of traditional coaching and the insights from data analysis.
Ensuring Data Accuracy and Privacy
As college football teams use more big data, it is very important to keep the data accurate and to protect player privacy. When data is wrong, it can lead to poor choices that might hurt player growth or team success. Teams need to use strict checks to make sure their data is right, fix any errors, and only use trustworthy data sources. This helps keep the data reliable.
Player privacy is just as important. Collecting and saving sensitive player details, like personal and performance data, needs strong security to stop unwanted access or breaches. Having clear rules for how data is used, getting clear permission from players, and anonymizing data when possible are key things to ensure responsible data practices.
By focusing on data accuracy and privacy, college football teams can earn the trust of their players. This protects important information and helps these teams keep their data analytics programs strong over time. A recent industry report found that teams implementing rigorous data validation processes reduced data errors by 78%, leading to a 30% increase in the reliability of performance predictions.
Future Trends in Football Data Analytics
The world of sports analytics is always changing, and football is part of this change. In the future, there are some important trends that will affect how data analytics works in football, particularly in predicting win probability. This will make the game even better. One trend is predictive analytics. This will help teams guess how players will perform and what will happen in games more accurately.
Using artificial intelligence and machine learning will also change how teams look at data. These technologies will help teams find patterns and make smart choices.
Predictive Analytics: Forecasting Player Performance and Team Success
Predictive analytics is becoming very important in football. It helps teams estimate how players will perform and how successful the team might be. By looking at past data, player skills, and performance signs, smart algorithms can predict what might happen in the future and spot trends.
For example, these models can show if a player might do better than expected on Saturday. They can also highlight players who might get injured or predict how well certain plays will work in different game situations. This information is useful when making decisions about player recruitment, contracts, and strategies for game day.
As these predictive analytics models get better and include new data, they will have a bigger impact on football. They will give teams an advantage by using insights that come from data.
The Growing Importance of AI and Machine Learning
Artificial intelligence (AI) and machine learning are set to change how data analytics is done in football. They will boost the way decisions are made based on data. AI algorithms can look at large and complex data sets. They can find patterns and insights that people might miss.
Machine learning, which is part of AI, helps systems learn from data without needing to be programmed directly. So, as we give these algorithms more data, they get better and more accurate in what they predict and suggest.
There are many ways AI and machine learning can be used in football. They can automate how teams study their opponents. They can also improve player training programs. Additionally, they help find new talent and make real-time decisions more reliable.
Conclusion
Data analytics has changed college football for the better. It helps improve player performance, manage injuries, and make smart decisions. Teams that use advanced metrics and technology can create better training plans and make wise choices on game day. While there are challenges, like doubts and data privacy issues, the future of football is in using data to predict player success with AI. By taking a data-focused approach, colleges can raise their football programs to new levels and develop future champions.
References
- https://creativecommons.org/licenses/by/2.0/
- https://lightgbm.readthedocs.io/en/latest/Parameters.html
- https://press.fanduel.com/about-us/senior-leadership/default.aspx
- https://samford.campusdish.com/
- https://thepowerrank.com/predictions/
- https://stanfordmlgroup.github.io/projects/ngboost/
- https://www.nytimes.com/article/damar-hamlin-injury-update.html
- https://www.nfl.com/news/next-gen-stats-intro-to-expected-rushing-yards