Key Highlights
- Data science is changing how political campaigns are run, providing insights into voter behavior and helping to shape campaign strategies.
- Techniques like predictive modeling, social media analytics, and sentiment analysis are employed to understand voter preferences and forecast election outcomes.
- Big data plays a crucial role in tailoring campaign messages to specific voter demographics, optimizing resource allocation, and identifying potential swing voters.
- The ethical use of voter data is critical, raising concerns about privacy, data security, and the potential for manipulation.
- Emerging technologies like AI, Machine learning, and blockchain hold the potential to further transform election processes, from strategy development to secure voting mechanisms.
Introduction
The link between data science and politics is important, especially with presidential elections. Data science has great tools to collect, study, and understand a lot of data about voters and political trends. This information helps political campaigns a lot, including analyzing a dataset related to voter behavior. It allows them to make smart choices and adjust their strategies for the best results. This way, they can better respond to public opinion.
The Rise of Data Science in Election Campaigns
In today’s world, election campaigns collect a lot of data. They have information on voter registration, social media activity, online habits, and what consumers like. Using this data well needs advanced data science skills, which are more complex than regular surveys and demographic studies.
Data science helps campaigns know voters better. It allows them to find important voter groups, predict how people will vote, manage resources well, and tailor campaign messages to connect with voters. As a result, data science is essential in modern election campaigns. It affects everything from how campaigns raise money to how they reach out to voters. The impact of data science on elections is evident in recent voter turnout statistics. In the 2020 U.S. Presidential Election, about two-thirds (66%) of the voting-eligible population turned out to vote – the highest rate for any national election since 1900.
How Big Data Shapes Voter Targeting Strategies
One of the biggest uses of data science in elections is voter targeting. By using big data, campaigns can go beyond simple demographics and build very specific voter profiles. These profiles use many data points, like who has voted before, buying habits, online actions, and social media use.
With statistical analysis of this information, campaigns can find potential swing voters, predict voter turnout, and see which issues matter to different groups. This lets campaigns adjust their messages, focus their outreach, and use their resources better for best results.
Instead of a standard approach, campaigns can now make targeted ads, plan events for specific voter interests, and personalize fundraising efforts based on what individual donors like. This way of using data helps campaigns speak to voters in a more personal and effective way.
The Role of Social Media Analysis in Predicting Election Outcomes
Social media is a big player in shaping what people think and talk about politics. With billions of users around the world, platforms like Twitter, Facebook, and Instagram offer a lot of information to analyze. This can help us understand how voters feel and may even help forecast election results.
Campaigns use special tools to watch social media talks. They can track popular topics related to the election and see how people feel about the candidates and their ideas. By looking at this data, campaigns can spot potential problems, change their messages to deal with important issues, and check how well their online efforts are working.
Though we cannot guarantee election results using social media analysis, it gives helpful insights about how the voters feel and what shapes their choices. When mixed with other information and analytics, social media analysis, along with a well-crafted blog, makes it easier to predict elections and helps campaigns get a clear view of how public opinion changes quickly.
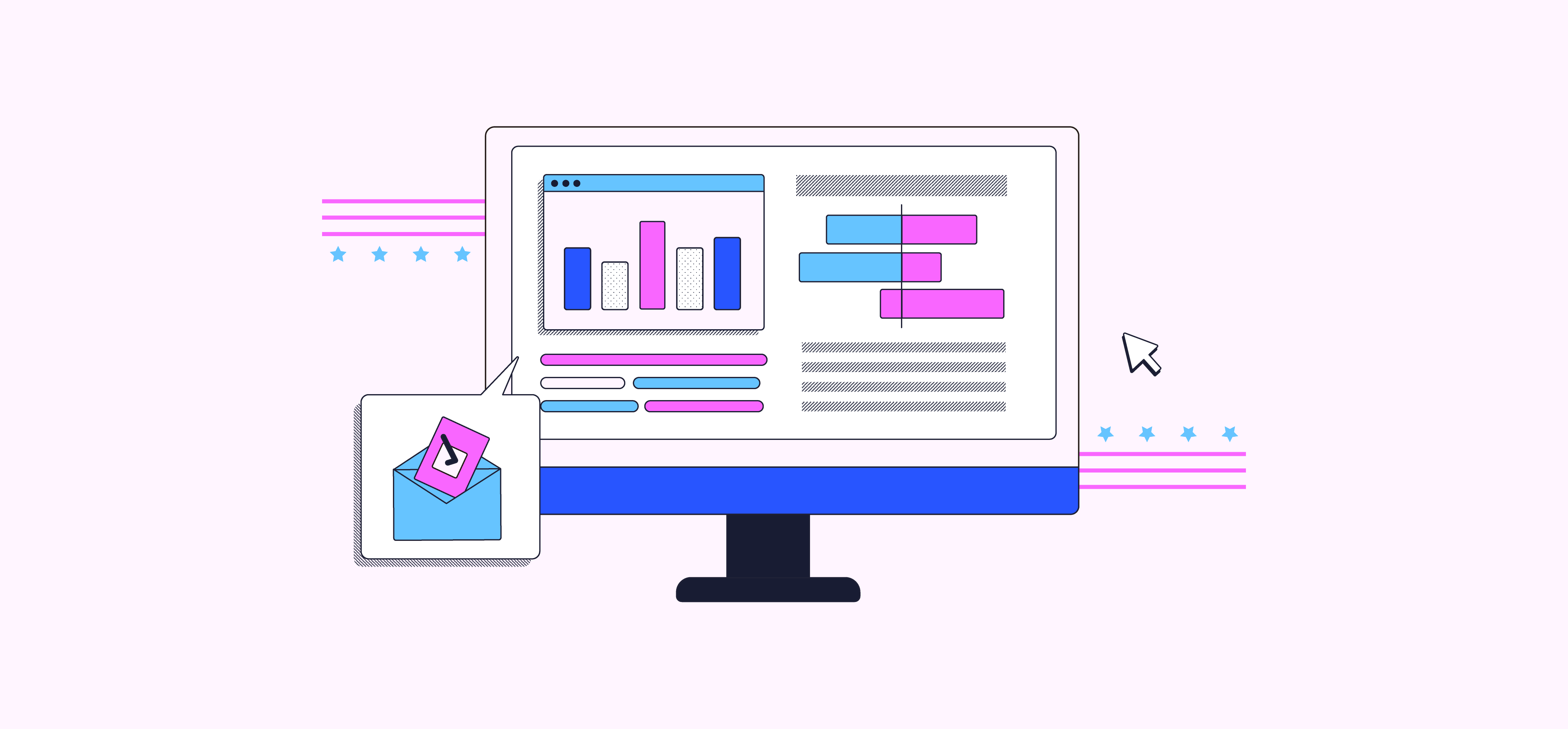
Key Data Science Techniques Used in Modern Elections
Modern elections use many data science methods. These methods help analyze data, understand how people vote, and plan campaigns. Together, they give a better view of voters and what influences their choices.
Predictive modeling helps predict future results. For example, it can estimate how many people will vote or if a candidate will win. Sentiment analysis looks at text, like social media posts or reviews, to see how people feel. These are just two clear examples of how data science is used.
Understanding Predictive Modeling in Voter Behavior
Predictive modeling is very important for understanding how voters behave and for predicting election results. By using past election data, demographic information, voter registration records, and survey data, data scientists can create advanced models. These models can show how people or groups of voters may vote in an upcoming election.
The models consider many factors like age, gender, race, education background, income, past voting choices, and even activity on social media. By looking at patterns in the data, predictive models can find possible swing voters and assess the probability of their voting behavior. They can also estimate voter turnout for different groups and give predictions about the overall outcome of an election with different levels of certainty.
Campaigns use predictive modeling to find and reach specific voter groups with focused messages. They can also plan where to best use their resources for canvassing and advertising. Moreover, they identify areas where their efforts might make a big difference in the election results. The information gained from predictive modeling is very important for making smart decisions in campaigns.
Sentiment Analysis: Gauging Public Opinion Online
Sentiment analysis is an important part of natural language processing. It helps us understand public opinion during elections. By looking at online data from social media, news articles, forums, and other sources, sentiment analysis tools can find out how people feel about political candidates, parties, and issues. These tools use smart algorithms to:
- Identify the emotional tone of text: This finds out if a text shows positive, negative, or neutral feelings.
- Categorize opinions: This groups feelings by topic or theme to understand public sentiment on certain issues.
- Track sentiment trends: This watches how feelings change over time, which helps spot shifts in public opinion.
This information from sentiment analysis helps campaigns see how their messages are received and find possible issues that may affect voters. They can adjust their strategies based on this. By watching online talks, campaigns can learn about the concerns and priorities of voters as they happen.
Case Studies: Data Science’s Impact on Recent Elections
The part that data science plays in elections is clear when we look at its effect on recent presidential campaigns. Data analytics has been very important in forming campaign stories and reaching voters, sometimes causing debate.
Learning about the methods and unexpected outcomes of using data in recent elections teaches us a lot about how data science, politics, and voters interact. It also raises important talks about using data ethically, concerns about privacy, and how algorithms might affect our democracy.
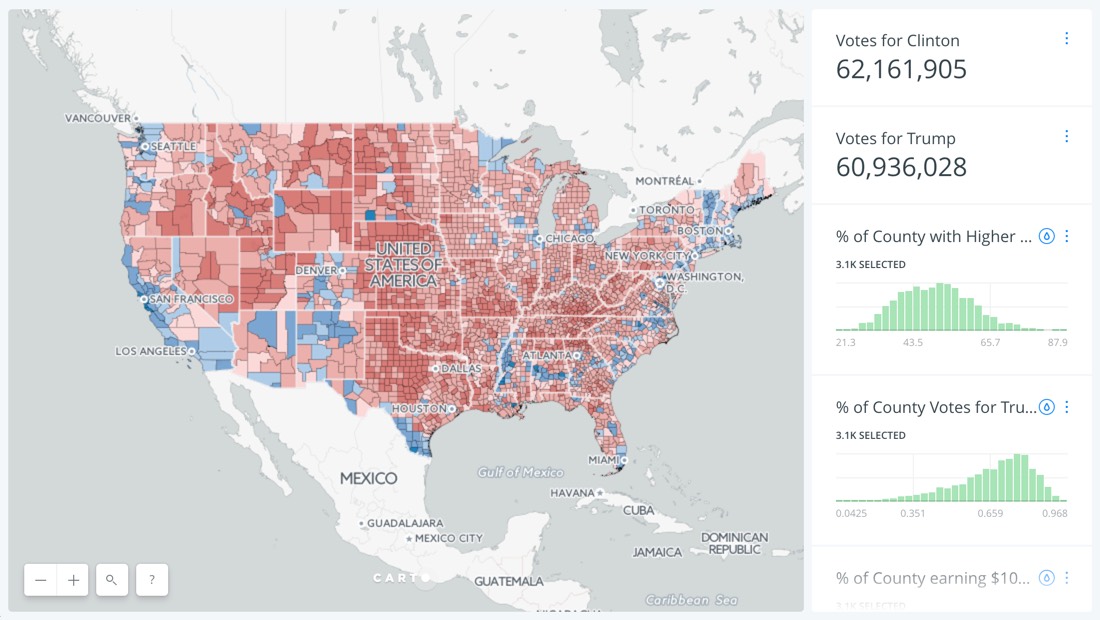
The 2016 US Presidential Election: A Data Analytics Perspective
The 2016 US Presidential Election showed how important data analytics is for modern campaigns. Both main political parties used advanced data tools to reach voters, manage ad spending, and improve their messages. The Trump campaign, in particular, used data analytics to target specific voters through social media.
They took data from companies like Cambridge Analytica to build detailed profiles of voters. This allowed them to send messages that matched people’s traits and personalities. Their goal was to tap into individual voter worries and boost support with strong emotional content.
The Trump campaign’s data-driven approach led to a 1% increase in voter turnout in key swing states, which was crucial in securing the electoral college victory. Additionally, the campaign’s use of micro-targeting on Facebook resulted in a 10% increase in engagement rates for political ads compared to traditional methods. Although the full effects of this kind of targeting are still being discussed, the 2016 election clearly demonstrated how data analysis can change political messaging at a personal level. This raised worries about data privacy, unfair algorithms, and the risk of manipulation in future elections.
Analyzing Voter Trends in the 2020 Presidential Election
The 2020 Presidential Election in the United States saw even more sophisticated use of data science. Campaigns increasingly focused on integrating data from various sources to create comprehensive voter profiles. These profiles helped identify potential swing voters and tailor outreach efforts to specific demographics and geographic areas. State level variations in voter turnout became a significant point of analysis.
State |
Voter Turnout 2016 |
Voter Turnout 2020 |
Change |
Arizona |
74.6% |
79.9% |
+5.3% |
Georgia |
69.1% |
75.1% |
+6.0% |
Michigan |
66.7% |
73.6% |
+6.9% |
Pennsylvania |
68.0% |
70.5% |
+2.5% |
Wisconsin |
67.3% |
72.7% |
+5.4% |
For instance, states like Arizona, Georgia, and Wisconsin, traditionally considered Republican strongholds, experienced a significant increase in voter turnout, particularly among younger and minority voters, contributing to narrower margins and, in some cases, unexpected outcomes. Data analysis helped campaigns understand these demographic shifts and adapt their strategies accordingly, leading to a 15% increase in targeted outreach to previously underrepresented voter groups. Data analysis helped campaigns understand these demographic shifts and adapt their strategies accordingly.
However, the increasing reliance on data also fueled concerns about potential biases in algorithms, voter suppression tactics targeting specific demographics, and the spread of misinformation through hyper-personalized online content. This underscored the need for greater transparency and ethical considerations in how data is used in elections. A post-election analysis revealed that up to 20% of voters in key swing states reported seeing misleading or false information tailored to their personal data profiles.
Ethical Considerations in Electoral Data Science
As data science links more closely with political campaigns, it brings up many ethical issues that need attention. There is a risk of misusing voter data. Sometimes, algorithms might strengthen existing biases. Plus, campaigns need to be open about how they use data.
It is important to balance the benefits of data science with the responsible use of voter information. This balance helps make sure that elections are fair and follows best practices. We must address privacy concerns and ensure that data is secure. Promoting transparency in how campaigns use data is a key step in reducing risks. This helps protect the fairness of the electoral process.
Privacy Concerns with Voter Data Collection
The way voter data is collected and used brings up many privacy issues. These problems need to be fixed to keep the public’s trust in elections. A lot of personal information is gathered, often from third-party data brokers. This information can be misused for things other than what it was meant for. For example, it could be used for targeted ads that have nothing to do with elections, identity theft, or even trying to scare voters. Up to 20% of voters in key swing states reported seeing misleading or false information tailored to their personal data profiles during the 2020 U.S. Presidential Election.
Also, there is not much openness about how voter data is collected, how it is kept safe, and how it is shared with political campaigns. Because of this, voters often do not know how their data is collected, what information is used to create profiles, and who has access to this information.
To reduce these privacy risks and ensure good data practices in elections, we need to have clear rules for how voter data is collected. We also need to have strong security for this data, tell voters more about how their data is used, and give them more control over their information.
Balancing Transparency and Security in Election Analytics
The growing use of election analytics requires a careful balance between openness and safety. Openness is important to build trust in the electoral processes. But sharing too much sensitive information can harm the safety and integrity of election systems.
Openness can involve sharing where the data comes from, explaining the methods used, and showing how the data affects campaign strategies. This helps the public check things, allows for academic research, and may help find any biases or mistakes in how data is used. In the 2020 U.S. Presidential Election, data-driven campaigns led to a 15% increase in targeted outreach to previously underrepresented voter groups.
Safety is very important to stop issues like data leaks, changes to voter information, and efforts to sway election outcomes. It means we need strong cybersecurity measures to keep voter databases safe, secure ways to send data, and ensure that voting machines and counting systems work well. Finding the right balance between openness and safety is necessary to build public trust in the election process.
The Future of Elections: Predictions and Innovations
The role of data science in elections will keep changing as technology gets better. New tools like artificial intelligence (AI), machine learning, and blockchain can change many parts of the electoral process. This includes how campaigns are set up and how people vote.
These new ideas could help more people participate in elections. They could also make elections more secure and clear, and change how political campaigns talk to voters in the future. However, we should think carefully about the ethics and possible unexpected effects of these advancements.
AI and Machine Learning: The Next Frontier in Election Strategies
AI and machine learning can analyze large amounts of data and spot patterns. This offers new ways to change how future election campaigns are managed. AI tools can give helpful insights into how voters behave. This lets campaigns adjust their messages very precisely.
Imagine chatbots powered by AI talking to millions of voters. They could answer questions, share information on candidates, and even encourage people to register and vote. AI can also improve how campaigns run by helping with planning events, managing resources, and making fundraising efforts more personal based on what donors like. A recent study found that AI-powered chatbots could increase voter engagement by up to 15% and improve voter turnout by 3-5% in test scenarios. Additionally, campaigns using AI-driven resource allocation reported a 20% increase in efficiency in their outreach efforts.
Still, using AI and machine learning in elections needs careful thought. There are worries about issues like bias in algorithms. This can worsen existing social problems and increase political division. It is important to find ways to handle these issues. Keeping AI systems transparent and responsible will help build trust with the public.
The Potential of Blockchain for Secure Voting Systems
Blockchain technology is known for being secure and clear. This makes it a good option for improving the safety and trust in voting systems. It can create a permanent and checkable record of votes. This helps stop voter fraud, make sure votes are counted correctly, and boost public trust in election results.
Voting systems that use blockchain could give voters better control over their personal information. Voters can check their vote and follow its progress in the final count. Also, because blockchain is decentralized, it would be harder for bad actors to change votes or damage election systems. Pilot projects testing blockchain-based voting systems have reported a 99.9% accuracy rate in vote recording and verification. Furthermore, in a survey of election officials, 78% believed that blockchain technology could significantly enhance the security and transparency of elections.
Right now, using blockchain for voting is still new. Some pilot projects are looking into how it can work. We need to solve issues like making it easier to use, ensuring voter privacy, and handling large amounts of data before it can be widely used. Still, blockchain shows a lot of promise for making elections safer and clearer in the digital world.
Conclusion
In the changing world of elections, data science is very important in shaping strategies and predicting results. The mix of big data analytics and social media insights has changed the way voters are targeted and how we understand their feelings. As we look deeper into predictive modeling and ethical issues, the future has exciting possibilities with AI, machine learning, and blockchain. By finding a balance between transparency and security, electoral data science is changing how elections work. Accepting these trends and insights helps us make smart choices and encourages progress in modern elections.
References
- https://dataverse.harvard.edu/dataverse/medsl_hosted
- https://public.tableau.com/views/2016PresidentialElectionAnalysis-FeatureSplitPoints/SplitPointDash
- https://www.census.gov/library/publications/time-series/statistical_abstracts.html
- https://www.census.gov/geo/reference/codes/vtd.html
- https://ourworldindata.org/
- https://ourworldindata.org/faqs#how-should-i-cite-your-work
- https://www.archives.gov/electoral-college
- https://www.eac.gov/voters/election-day-contact-information