Key Highlights
- A data scientist resume should highlight technical skills, relevant experience, and impactful projects.
- Tailor your resume to each job description, emphasizing keywords and desired qualifications.
- Showcase your achievements using metrics and quantifiable results to demonstrate your impact.
- Structure your resume for clarity and readability, using a chronological format and concise language.
- Highlight both technical expertise and soft skills, such as communication and teamwork, to showcase your versatility.
Introduction
A good data scientist resume is key for getting a job. It is the first thing employers see and shows your skills, experience, and love for data science. You should highlight your technical skills and relevant work experience. Make sure to include your contact information clearly. Your data scientist resume should tell a strong story about your qualifications. Tailor it to each job description to leave a great impression. According to the U.S. Bureau of Labor Statistics, data scientist positions are projected to grow by 35% from 2022 to 2032, much faster than the average for all occupations. Additionally, the World Economic Forum’s Future of Jobs 2023 report estimates that by 2027, the demand for AI and machine learning specialists will increase by 40%, and for data analysts, scientists, engineers, BI analysts, and other big data and database professionals will grow by 30%–35%. With an average salary range of $160,000–$200,000 annually, data science continues to be a lucrative career path.
Understanding the Role of a Data Scientist
A data scientist is a professional that many companies want to hire. They have a special mix of technical skills and business knowledge. Their main job is to get valuable insights from data. They use their skills in data analysis, machine learning, and statistical modeling to tackle tough business issues.
Data scientists are vital in shaping business plans. They find patterns, predict future trends, and give data-based advice. This advice helps companies make better choices. Their skill to connect basic data and useful insights is important for businesses that want to use data for growth and new ideas.
The Intersection of Data Analysis, Machine Learning, and Business Strategy
Data analysis is very important for a data scientist. It means looking carefully at raw data to find patterns. Then, the data can be changed into helpful information that is easy to understand and use for making decisions. This work often uses different methods, data visualization tools, and programming languages like Python or R.
Machine learning is a part of artificial intelligence. Data scientists use it as a strong tool. They create algorithms and models that can learn from data. This helps them automate tasks, make predictions, and find insights that may be hard to see with regular methods. In 2024, machine learning skills are mentioned in 69% of job postings, underscoring their critical importance.
The real benefits of data analysis and machine learning show up when they help a business strategy. By matching data insights with important business goals, like improving customer experience or making marketing campaigns better, data scientists help drive business growth. It can also support innovation and offer a competitive edge.
Key Competencies and Skills for Today’s Data Scientists
The world of data science keeps changing, so people wanting to be data scientists need to have many technical skills. Knowing programming languages like Python and R is very important. These skills help with data manipulation, analysis, and building models.
Besides programming, being good with data visualization tools like Power BI and Tableau is also key. These tools help turn complex data into simple charts and graphs. This ability is important for sharing findings with others.
It’s also critical to have a strong grasp of statistics and machine learning methods. These technical skills help data scientists make accurate predictions, spot trends, and create models that address real business challenges.
- Key programming languages: Python, R, SQL
- Data visualization tools: Tableau, Power BI
- Machine learning libraries: scikit-learn, TensorFlow
Preparing to Craft Your Data Scientist Resume
Before you begin writing your data scientist resume, you should collect all important work experience, education details, and information about projects you have done. This will help you show a complete view of your qualifications.
Review the requirements in each job description carefully. Make sure to adjust your resume to match what they are looking for. By highlighting the specific skills and experiences that fit the job, you can boost your chances of standing out from other applicants. According to a survey by Zippia, 63% of recruiters prefer resumes tailored to the open position, emphasizing the importance of customization.
Gathering Necessary Information and Resources
- Start by gathering your correct and current contact information. This should include your full name, phone number, professional email address, and location. You may want to add links to your online profiles, like LinkedIn and GitHub, to show your professional network and project portfolio. Research shows that 87% of recruiters use LinkedIn to vet candidates during the hiring process.
- Next, collect details about your education. List any degrees or certifications you have, along with the institutions you attended and when you graduated. Make sure to highlight any relevant classes or academic projects that show your data science knowledge and skills. A study by the National Association of Colleges and Employers found that 80.7% of employers screen candidates based on their GPA.
- Lastly, create a complete list of your technical skills, software you know, and programming languages. This should include tools like Python, R, and SQL, as well as any experience you have with data visualization software. According to the 2021 Kaggle Machine Learning and Data Science Survey, Python is the most popular programming language among data scientists, with 87% of respondents using it.
Analyzing Job Descriptions to Tailor Your Resume
It is very important to look closely at the job description before you write your data scientist resume. Focus on the skills, qualifications, and duties listed in the job post.
Find your most relevant work experience and change it to fit the job needs. Show projects and success stories that prove you can use your skills effectively.
By customizing your resume for each job description, you let the employer know you understand what they want. This also shows that your qualifications match the job, which can boost your chances of being noticed. A study by Ladders found that recruiters spend an average of 7.4 seconds reviewing a resume, highlighting the need for a targeted and impactful document.
Essential Components of a Data Scientist Resume
A data scientist resume needs to have important sections that show your qualifications clearly. Begin with a strong summary or objective statement that highlights your skills and experience. In the work experience section, describe your past roles. Focus on what you achieved and how you contributed.
Make sure to include a focused skills section. List your technical skills, such as programming languages, data analysis tools, and machine learning algorithms. This will show your expertise to potential employers. According to a survey by CareerBuilder, 70% of employers spend less than five minutes reviewing a resume, highlighting the importance of a well-structured and concise document.
Crafting an Engaging Professional Summary
A good resume summary is important for catching the hiring manager’s eye and making a strong first impression. It should briefly show your best skills, experience, and goals as a data scientist.
Make sure your resume summary fits the specific job you want. Point out keywords and skills that match what they are looking for. Use action verbs and clear results from your past jobs to show your value to potential employers.
Remember, your resume summary shows who you are as a data scientist. It should be clear and interesting. This way, you can share your value and make the hiring manager want to find out more about you.
Highlighting Relevant Work Experience
Your work experience section should show your past jobs and successes as a data scientist. List your jobs starting from the most recent one. For each job, give a short summary of what you did. Use strong action words to show what you achieved.
Focus on results that can be measured. Use numbers and data to display how you made a difference in your past jobs. This could include things like improving model accuracy, boosting customer engagement, or cutting costs. For example, a study by LinkedIn found that including quantifiable achievements can increase the likelihood of getting noticed by up to 40%.
- Created and used a machine learning model that improved customer churn prediction by 15%.
- Carried out A/B tests on marketing campaigns, which led to a 10% rise in click-through rates.
- Worked with different teams to study customer data and find important insights for product development.
Detailing Your Educational Background
In the education section of your data scientist resume, give a clear summary of your academic history. List your degrees starting with the most recent one. Include the degree name, your major, the school you attended, and when you graduated for each one.
If you have a degree in a related field like data science, computer science, or statistics, mention any important classes or projects that show your knowledge. If your degree is in a different area, focus on any classes or training in data science or similar fields.
For those who just graduated, think about including your GPA if it is over 3.5. You can also share any honors, awards, or scholarships you earned to make your qualifications stand out more. According to the National Association of Colleges and Employers, 81% of employers screen candidates based on their GPA, making it a relevant detail to include if it is strong.
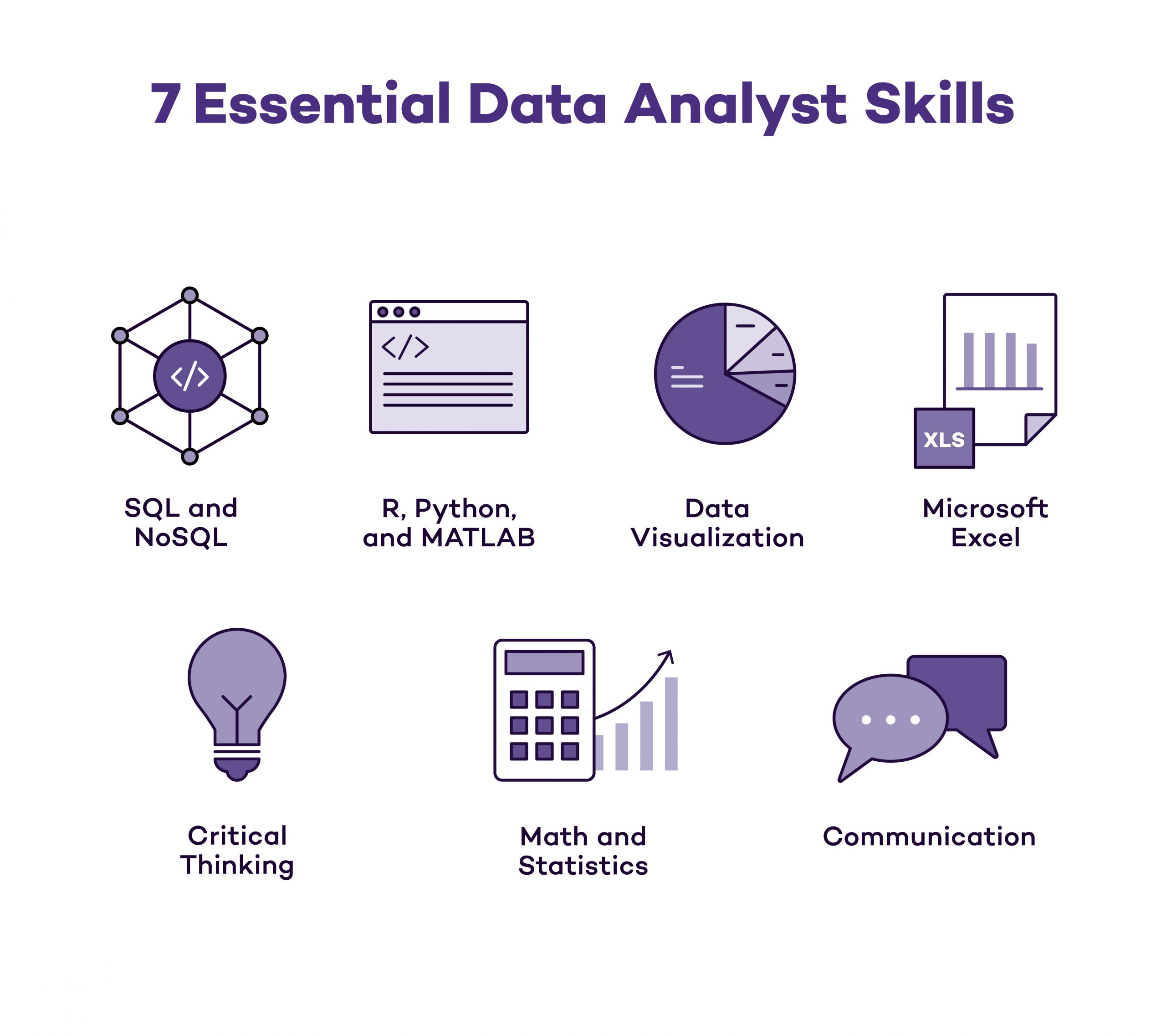
Showcasing Your Technical Skill Set
In the competitive area of data science, it is important to show your technical skills. Make a special section on your resume that includes your skills in programming languages, data analysis tools, and data visualization software.
List programming languages like Python, R, and SQL. Be sure to mention any special libraries or packages you know. Also, include data visualization tools like Tableau, Power BI, or matplotlib to show how you can share data insights clearly. According to the 2021 Kaggle Machine Learning and Data Science Survey, 87% of data scientists use Python, making it the most popular programming language in the field.
Key Programming Languages and Tools for Data Science
Proficiency in programming languages is very important for people who want to become data scientists. Python is a popular option. It has many libraries like Pandas and scikit-learn. These tools are great for data manipulation, analysis, and machine learning.
R is another strong language. It has useful statistical packages and tools for data visualization. Many use R in schools and businesses. SQL is also key for working with relational databases. It helps data scientists get and manage data for their analysis. A survey by DataCamp found that 85% of data scientists use SQL regularly, highlighting its importance in the field.
These programming languages, plus visualization tools like Tableau and Power BI, help data scientists change raw data into valuable insights. These insights can help make business decisions.
Projects and Portfolios: Demonstrating Your Expertise
In addition to having technical skills, it is very important to show your experience with data science projects. You should add a portfolio section to your resume. In this section, highlight specific projects where you used your skills to solve real problems.
If you have a GitHub profile, make sure to include the link. This will show your code, projects, and contributions to the data science community. When you talk about your projects, clearly mention the problem you aimed to solve, the methods you used, and what you achieved. A study by Dice found that 79% of hiring managers consider a candidate’s GitHub profile when evaluating their technical skills.
- Developed a sentiment analysis model to sort customer reviews as positive, negative, or neutral. This helped improve response time in customer service.
- Built a recommendation engine using collaborative filtering. This suggests products to users based on what they browsed, increasing sales by 5%.
- Created a data visualization dashboard to monitor key performance indicators for a marketing campaign. It gave the team useful insights.
A Guide to Structuring Your Data Scientist Resume
A good data scientist resume helps the hiring manager see your skills clearly. Use a simple and direct format, putting your work experience and education in chronological order.
Begin with your contact information. Then, write a strong summary or objective statement. Focus on your work experience, showing measurable successes and relevant skills. Have a section just for your technical skills. This should show your knowledge of programming languages, tools, and technologies.
Choosing the Right Format: Chronological vs. Functional
Choosing the right resume format is very important for showing your qualifications well. The most common format for data scientist resumes is the chronological resume. This format lists your work history from most recent to oldest. It shows your career growth and achievements over time.
Another option is the functional resume. This type focuses on your skills and abilities instead of your work history. It can work well for people who have gaps in their jobs or are switching fields. Still, for most data scientist jobs, a chronological resume is better. It gives a clear view of your career path and achievements. A study by TheLadders found that recruiters spend an average of 7.4 seconds reviewing a resume, so placing your most relevant information near the top is crucial.
No matter which format you pick, make sure your resume is easy to read, clean, and matches the job description you want.
Organizing Sections for Maximum Impact
Organizing your resume sections strategically can significantly enhance its impact and make it easier for hiring managers to assess your qualifications. Start with your contact information, followed by a compelling summary or objective statement that highlights your key skills and experiences.
Next, prioritize your work experience, listing your roles in reverse-chronological order. For each position, provide a brief description of your responsibilities, emphasizing quantifiable achievements and relevant skills. Follow with your education section, highlighting relevant coursework or academic projects.
Finally, dedicate a section to showcase your technical skills. List programming languages, data analysis tools, and other relevant technologies you are proficient in. This section should be concise and easy to scan. According to a CareerBuilder survey, 61% of recruiters will automatically dismiss a resume that is not customized to the job.
Example Resume Section Order:
Section |
Description |
Contact Information |
Full name, phone number, email address, location, LinkedIn profile URL |
Summary/Objective |
Concise overview of your skills, experiences, and career goals |
Work Experience |
Reverse chronological order of previous roles, highlighting quantifiable achievements and relevant skills |
Education |
Degrees obtained, major, institution attended, graduation dates, relevant coursework |
Skills |
Technical proficiencies, programming languages, data analysis tools, and visualization software |
Projects/Portfolio |
Showcase practical experience through specific projects |
How to Begin Crafting Your Resume: A Step-by-Step Approach
Start creating your data scientist resume by picking a clear and neat template. Make sure your resume is easy to read and well-arranged. Use keywords from the job description in your resume. This helps it reach a hiring manager by getting past applicant tracking systems (ATS).
Use action verbs to explain your achievements. Whenever you can, include numbers to show your success. This shows your impact and gives clear proof of your skills and achievements.
Step 1: Start With a Strong Foundation
Start your data scientist resume with clear and up-to-date contact information. This should include your full name, phone number, a professional email address, and your location. Make sure your email looks professional and includes your name or initials.
Then, create a strong resume summary. This should show your most important skills, work experience, and career goals. Tailor this summary to match the job description. Use keywords and qualifications that fit the role.
Keep in mind that your resume summary acts like your elevator pitch to the hiring manager. It should be clear, brief, and engaging. This way, you make a great first impression and get them to want to know more about you.
Step 2: Drafting Your Professional Experience
When you write about your work experience, start with your most recent job. List your past roles in reverse order. Include the company name, your job title, and the dates you worked there for each job.
Focus on what you achieved in your roles. Don’t just write down your duties. Use action verbs to explain what you did. If possible, include numbers to show your success. This can help show your skills and the impact you made.
If you are a recent graduate or don’t have much work experience, include internships, volunteer work, or school projects. These can show your skills in data science. Remember, any related experience can improve your resume.
Step 3: Incorporating Your Educational Achievements
Your education section should give a clear picture of your school background. Start with your highest degree. Write down the degree name, your major, where you studied, and when you graduated for each item.
Mention any important classes, research, or accomplishments that show your knowledge and skills in data science or related areas. If you have done any online courses, bootcamps, or workshops, think about adding a section for certifications. This will show your commitment to learning in this field.
Keep in mind, your education section should not only show your school credentials. It should also highlight your love for data science and your ongoing effort to grow your knowledge and skills.
Step 4: Highlighting Your Skills and Projects
- Create a special section on your resume to show your technical skills and strengths.
- List the programming languages and data analysis tools you know.
- Include other relevant technologies you have used.
- Mention both the skills you are good at and those you are still learning.
- Also, highlight your project portfolio.
- Give a brief description of the data science projects you have worked on.
- Focus on the problem you solved, the methods you used, and the results you got.
- By showing your technical skills and project portfolio, you prove your real-life experience.
- This helps you apply your knowledge to actual problems, making your resume more attractive to future employers.
Step 5: Polishing and Proofreading
- After finishing your data scientist resume, take time to check it carefully. Make sure there are no grammar mistakes, typos, or formatting issues.
- Use bullet points to share information in a clear and simple way. Add white space to make your resume look nice and avoid any clutter. These details help to make your resume more professional.
- Before sending your resume, have a friend, colleague, or mentor look it over. Fresh eyes can find mistakes you may not see. A well-structured, error-free resume is important for making a good first impression on employers.
Advanced Tips for a Stand-Out Data Scientist Resume
To make your data scientist resume stand out in a crowded job market, use important keywords from the job description. Show off your technical skills, software knowledge, and industry terms. This helps your resume match what the job needs.
Whenever you can, add numbers to show your achievements. Instead of saying “improved” or “increased,” give clear data that shows the results of your work.
Leveraging Keywords from Job Postings
When you apply for a data science job, take a close look at the job description. It helps you find important keywords. These words show the skills, qualifications, and experience employers want in a candidate.
Use these keywords in your resume. Focus on your summary, skills section, and work experience descriptions. Using the same words as the job post shows that you understand the job. It also makes your profile match what they need.
If you adjust your resume for each data science job you apply to, you have a better chance of being noticed. This works for both applicant tracking systems (ATS) and hiring managers. It makes you stand out among other candidates. A survey by JobScan found that 98.8% of Fortune 500 companies use applicant tracking systems (ATS), which scan resumes for relevant keywords.
Including Metrics to Quantify Achievements
Numbers are important in the world of data science. They can show your success more clearly than just words. When you share your achievements, try to use specific numbers and data. For instance, instead of saying you made a model better, say, “I improved model accuracy by 10%, which helped keep customers by 5% more.”
Including numbers in your data scientist resume helps prove your skills and experience. It also shows that you can think analytically and make sense of data. Recruiters like to see clear results and numbers that show what you have done.
When you use numbers to describe your work, you give a better idea of what you can do. This helps show how much value you can add to their team. It’s all about proving you can get real results with data-driven insights.
Avoiding Common Pitfalls in Data Scientist Resumes
While showing your technical skills is important, don’t forget to mention soft skills in your data scientist resume. It’s good to highlight your communication, teamwork, and problem-solving skills. These are very important in data science jobs where people work together.
Do not use the same resume for every job you apply for. Change your resume to match each job by showing relevant work experience, skills, and projects that fit the job description and requirements.
Overlooking the Importance of Soft Skills
Data science is more than just working with numbers and creating algorithms. It also needs strong soft skills to share insights and work well with others. Employers want people who can connect technical skills with business knowledge.
Showcase your communication skills by explaining complex data topics in simple terms. Provide examples that highlight your teamwork by sharing successful projects with coworkers, clients, or stakeholders.
Highlight your problem-solving skills too. Talk about how you faced challenges, found new solutions, and delivered results with your data science skills. By adding soft skills to your data scientist resume, you show that you are a complete candidate. You can handle technical tasks and also do well in teamwork and fast-paced work settings.
Failing to Tailor Your Resume for the Role
One common mistake that people applying for data science jobs make is using the same resume for every job. Hiring managers can easily spot resumes that are not personalized. These generic resumes are less likely to get noticed.
Before you send your resume, take time to look at the job description. Find the specific skills, experience, and projects that match the job requirements. Change your resume summary to show the qualifications mentioned in the posting.
By tailoring your resume for each job application, you show potential employers that you understand what they need. You also show that your qualifications fit their requirements. This personal touch can greatly improve your chances of standing out among other applicants.
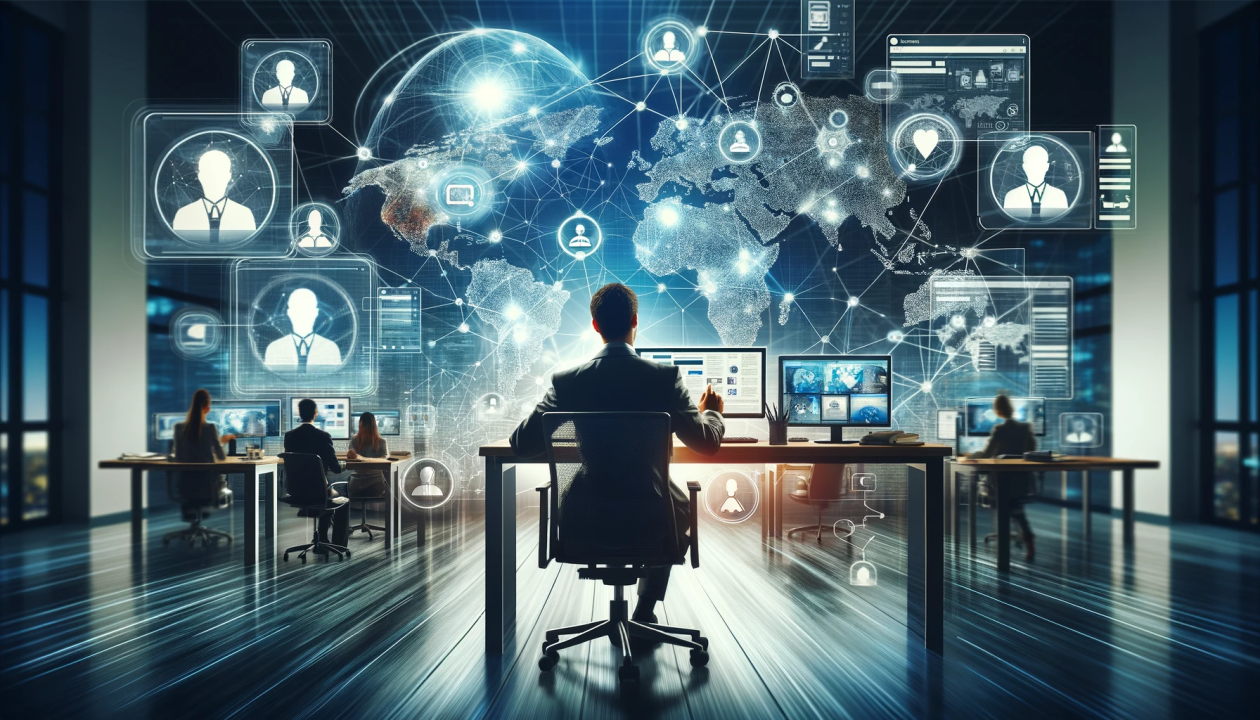
The Role of Networking and Online Presence
In today’s connected world, networking and being online are very important for your job search. You should build your network by going to industry events, joining online groups, and talking to people who work in the data science field.
Having a strong LinkedIn profile can really help you online. Make sure to improve your profile using relevant keywords. Show your experience and skills, share helpful content, and connect with others in your field.
Utilizing LinkedIn and Other Professional Networks
LinkedIn is a strong platform for people in the field of data science. It helps you connect with others, show your skills, and find job offers. Make sure your LinkedIn profile is up-to-date and includes all your experience, skills, and projects.
Join and take part in LinkedIn groups that focus on data science. Join discussions and share articles or insights that are important. By building a good professional network on LinkedIn, you can find new job chances and grow your presence in the data science community.
Besides LinkedIn, look into other professional networks and online sites that relate to your field. Go to industry conferences, join webinars, and get involved in online forums. This can help you be seen more and give chances for networking and growing in your profession.
Engaging with the Data Science Community Online
The data science community is lively and busy online. It offers many chances for learning, working together, and growing. You can join online forums and communities like Stack Overflow or Data Science Central. There, you can talk with others, ask for help, and share your ideas.
You can improve your knowledge and skills by taking online courses or bootcamps. Platforms like DataCamp or Coursera have structured lessons, hands-on projects, and recognized certifications that can boost your credentials.
Think about sharing what you know. You can write blog posts, make tutorials, or help with open-source projects. This sharing can raise your profile and help you become a thought leader in the data science field.
Preparing for the Job Hunt: Next Steps After Your Resume
After you make a strong data scientist resume, write a cover letter. This letter should show your interest in specific jobs and how your skills fit the job needs.
Practice interview skills. Focus on technical questions and situations you might face. Talk clearly about your experiences and achievements. Getting ready for data science job interviews shows you really want the opportunity.
Crafting a Compelling Cover Letter
A strong cover letter is important for your data scientist resume. It provides a chance to explain your skills and experiences in detail. You can show your passion for data science and why you are a great match for the job.
Start the letter by addressing it to the hiring manager or recruiter. Make it personal whenever you can. Change the content to meet the specific needs mentioned in the job description. Show that you understand the company’s mission and values. Explain how your skills and experiences match their goals.
Point out your key accomplishments and projects. Share how you solved real problems using data science methods. Let them know you are excited to join their team and are dedicated to achieving meaningful results with data-driven insights.
Strategies for Navigating Data Science Job Interviews
Data science job interviews are complex. They examine your technical skills, problem-solving, and how well you fit into the company. You should get ready for technical questions about programming languages, algorithms, data structures, and statistical ideas.
Practice explaining your thought process clearly and simply. Employers want to see how you solve problems and explain hard technical ideas. Learn about the company’s work, trends in the industry, and the challenges they may face.
Be ready to talk about your past projects. Focus on what you did, the challenges you tackled, and the results you got. Having a thoughtful chat with the data science manager or team shows your passion, knowledge, and how you can help the company.
Conclusion
Crafting a strong resume for a data scientist requires a mix of skills and experience in the field of data science. Start by knowing what to include in your resume. Make sure to structure it well and show off your technical skills and project achievements. This way, you will stand out in a tough job market. Each time, adjust your resume to match the job description. Use important keywords and provide numbers to back up your accomplishments.
As you start your job search, focus on networking with others, improving your online presence, and getting ready for interviews. This will help you show your skills and passion for data science. With a good resume and an active approach, you can become a sought-after data scientist in the industry.
References
- https://medium.com/business
- https://www.coursera.org/learn/machine-learning
- https://www.sas.com/en_th/certification/credentials/data-management/big-data-professional.html
- https://www.sas.com/en_us/certification/credentials/advanced-analytics/advanced-analytics-professional.html
- https://www.quora.com/Will-data-science-analysis-jobs-be-oversaturated
- https://pll.harvard.edu/series/professional-certificate-data-science
- https://resume.io
- https://skillslash.com/data-science-course
- https://speechify.com/medium
- https://towardsdatascience.com/breaking-into-data-science-in-2021-is-it-still-easy-as-before-9bb6fe313a0f
- https://www.bls.gov/opub/btn/volume-7/big-data-adds-up.htm